- 5.1 Introduction
- 5.2 Loss Functions
- 5.3 Machine Learning via Bayesian Methods
- 5.4 Conclusion
5.2 Loss Functions
We introduce what statisticians and decision theorists call loss functions. A loss function is a function of the true parameter, and an estimate of that parameter

The important point of loss functions is that they measure how bad our current estimate is: The larger the loss, the worse the estimate is according to the loss function. A simple, and very common, example of a loss function is the squared-error loss, a type of loss function that increases quadratically with the difference, used in estimators like linear regression, calculation of unbiased statistics, and many areas of machine learning

The squared-error loss function is used in estimators like linear regression, calculation of unbiased statistics, and many areas of machine learning. We can also consider an asymmetric squared-error loss function, something like:
which represents that estimating a value larger than the true estimate is preferable to estimating a value that is smaller. A situation where this might be useful is in estimating Web traffic for the next month, where an overestimated outlook is preferred so as to avoid an underallocation of server resources.
A negative property about the squared-error loss is that it puts a disproportionate emphasis on large outliers. This is because the loss increases quadratically, and not linearly, as the estimate moves away. That is, the penalty of being 3 units away is much less than being 5 units away, but the penalty is not much greater than being 1 unit away, though in both cases the magnitude of difference is the same:

This loss function implies that large errors are very bad. A more robust loss function that increases linearly with the difference is the absolute-loss, a type of loss function that increases linearly with the difference, often used in machine learning and robust statistics.

Other popular loss functions include the following.
is the zero-one loss often used in machine-learning classification algorithms.
,
, 1, θ
[0, 1], called the log-loss, is also used in machine learning.
Historically, loss functions have been motivated from (1) mathematical ease and (2) their robustness to application (that is, they are objective measures of loss). The first motivation has really held back the full breadth of loss functions. With computers being agnostic to mathematical convenience, we are free to design our own loss functions, which we take full advantage of later in this chapter.
With respect to the second motivation, the above loss functions are indeed objective in that they are most often a function of the difference between estimate and true parameter, independent of positivity or negativity, or payoff of choosing that estimate. This last point—its independence of payoff—causes quite pathological results, though. Consider our hurricane example: The statistician equivalently predicted that the probability of the hurricane striking was between 0% and 1%. But if he had ignored being precise and instead focused on outcomes (99% chance of no flood, 1% chance of flood), he might have advised differently.
By shifting our focus from trying to be incredibly precise about parameter estimation to focusing on the outcomes of our parameter estimation, we can customize our estimates to be optimized for our application. This requires us to design new loss functions that reflect our goals and outcomes. Some examples of more interesting loss functions include the following.
,
, θ
[0, 1] emphasizes an estimate closer to 0 or 1, since if the true value θ is near 0 or 1, the loss will be very large unless
is similarly close to 0 or 1. This loss function might be used by a political pundit who’s job requires him or her to give confident “Yes/No” answers. This loss reflects that if the true parameter is close to 1 (for example, if a political outcome is very likely to occur), he or she would want to strongly agree so as to not look like a skeptic.
is bounded between 0 and 1 and reflects that the user is indifferent to sufficiently-far-away estimates. It is similar to the zero-one loss, but not quite as penalizing to estimates that are close to the true parameter.
Complicated non-linear loss functions can programmed:
def loss(true_value, estimate): if estimate*true_value > 0: return abs(estimate - true_value) else: return abs(estimate)*(estimate - true_value)**2
- Another example in everyday life is the loss function that weather forecasters use. Weather forecasters have an incentive to report accurately on the probability of rain, but also to err on the side of suggesting rain. Why is this? People much prefer to prepare for rain, even when it may not occur, than to be rained on when they are unprepared. For this reason, forecasters tend to artificially bump up the probability of rain and report this inflated estimate, as this provides a better payoff than the uninflated estimate.
5.2.1 Loss Functions in the Real World
So far, we have been acting under the unrealistic assumption that we know the true parameter. Of course, if we know the true parameter, bothering to guess an estimate is pointless. Hence a loss function is really only practical when the true parameter is unknown.
In Bayesian inference, we have a mindset that the unknown parameters are really random variables with prior and posterior distributions. Concerning the posterior distribution, a value drawn from it is a possible realization of what the true parameter could be. Given that realization, we can compute a loss associated with an estimate. As we have a whole distribution of what the unknown parameter could be (the posterior), we should be more interested in computing the expected loss given an estimate. This expected loss is a better estimate of the true loss than comparing the given loss from only a single sample from the posterior.
First, it will be useful to explain a Bayesian point estimate. The systems and machinery present in the modern world are not built to accept posterior distributions as input. It is also rude to hand someone over a distribution when all they asked for was an estimate. In the course of our day, when faced with uncertainty, we still act by distilling our uncertainty down to a single action. Similarly, we need to distill our posterior distribution down to a single value (or vector, in the multivariate case). If the value is chosen intelligently, we can avoid the flaw of frequentist methodologies that mask the uncertainty and provide a more informative result. The value chosen, if from a Bayesian posterior, is a Bayesian point estimate.
If P(θ|X) is the posterior distribution of θ after observing data X, then the following function is understandable as the expected loss of choosing estimate to estimate θ:
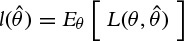
This is also known as the risk of estimate . The subscript θ under the expectation symbol is used to denote that θ is the unknown (random) variable in the expectation, something that at first can be difficult to consider.
We spent all of Chapter 4 discussing how to approximate expected values. Given N samples θi, i = 1, ..., N from the posterior distribution, and a loss function L, we can approximate the expected loss of using estimate by the Law of Large Numbers:

Notice that measuring your loss via an expected value uses more information from the distribution than the MAP estimate—which, if you recall, will only find the maximum value of the distribution and ignore the shape of the distribution. Ignoring information can overexpose yourself to tail risks, like the unlikely hurricane, and leaves your estimate ignorant of how ignorant you really are about the parameter.
Similarly, compare this with frequentist methods, that traditionally only aim to minimize the error, and do not consider the loss associated with the result of that error. Compound this with the fact that frequentist methods are almost guaranteed to never be absolutely accurate. Bayesian point estimates fix this by planning ahead: If your estimate is going to be wrong, you might as well err on the right side of wrong.
5.2.2 Example: Optimizing for the Showcase on The Price Is Right
Bless you if you are ever chosen as a contestant on The Price Is Right, for here we will show you how to optimize your final price on the Showcase. For those who don’t know the rules:
- Two contestants compete in the Showcase.
- Each contestant is shown a unique suite of prizes.
- After the viewing, the contestants are asked to bid on the price for their unique suite of prizes.
- If a bid price is over the actual price, the bid’s owner is disqualified from winning.
- If a bid price is under the true price by less than $250, the winner is awarded both prizes.
The difficulty in the game is balancing your uncertainty in the prices, keeping your bid low enough so as to not bid over, and to bid close to the price.
Suppose we have recorded the Showcases from previous The Price Is Right episodes and have prior beliefs about what distribution the true price follows. For simplicity, suppose it follows a Normal:
- True Price ~ Normal(μp, σp)
For now, we will assume μp = 35,000 and σp = 7,500.
We need a model of how we should be playing the Showcase. For each prize in the prize suite, we have an idea of what it might cost, but this guess could differ significantly from the true price. (Couple this with increased pressure from being onstage, and you can see why some bids are so wildly off.) Let’s suppose your beliefs about the prices of prizes also follow Normal distributions:
- Prizei ~ Normal(μi, σi), i = 1, 2
This is really why Bayesian analysis is great: We can specify what we think a fair price is through the μi parameter, and express uncertainty of our guess in the σi parameter. We’ll assume two prizes per suite for brevity, but this can be extended to any number. The true price of the prize suite is then given by Prize1 + Prize2 + , where
is some error term. We are interested in the updated true price given we have observed both prizes and have belief distributions about them. We can perform this using PyMC.
Let’s make some values concrete. Suppose there are two prizes in the observed prize suite:
- A trip to wonderful Toronto, Canada!
- A lovely new snowblower!
We have some guesses about the true prices of these objects, but we are also pretty uncertain about them. We can express this uncertainty through the parameters of the Normals:
Snowblower ~ Normal(3000, 500)
Toronto ~ Normal(12000, 3000)
For example, I believe that the true price of the trip to Toronto is 12,000 dollars, and that there is a 68.2% chance the price falls 1 standard deviation away from this; that is, my confidence is that there is a 68.2% chance the trip is in [9000, 15000]. These priors are graphically represented in Figure 5.2.1.
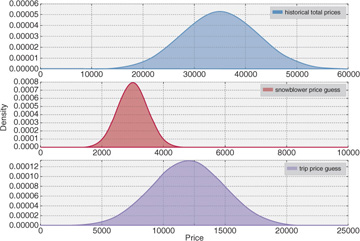
Figure 5.2.1: Prior distributions for unknowns: the total price, the snowblower’s price, and the trip’s price
We can create some PyMC code to perform inference on the true price of the suite, as shown in Figure 5.2.2.
%matplotlib inline
import scipy.stats as stats
from IPython.core.pylabtools import figsize
import numpy as np
import matplotlib.pyplot as plt
plt.rcParams['savefig.dpi'] = 300
plt.rcParams['figure.dpi'] = 300
figsize(12.5, 9)
norm_pdf = stats.norm.pdf
plt.subplot(311)
x = np.linspace(0, 60000, 200)
sp1 = plt.fill_between(x, 0, norm_pdf(x, 35000, 7500),
color="#348ABD", lw=3, alpha=0.6,
label="historical total prices")
p1 = plt.Rectangle((0, 0), 1, 1, fc=sp1.get_facecolor()[0])
plt.legend([p1], [sp1.get_label()])
plt.subplot(312)
x = np.linspace(0, 10000, 200)
sp2 = plt.fill_between(x, 0, norm_pdf(x, 3000, 500),
color="#A60628", lw=3, alpha=0.6,
label="snowblower price guess")
p2 = plt.Rectangle((0, 0), 1, 1, fc=sp2.get_facecolor()[0])
plt.legend([p2], [sp2.get_label()])
plt.subplot(313)
x = np.linspace(0, 25000, 200)
sp3 = plt.fill_between(x, 0, norm_pdf (x, 12000, 3000),
color="#7A68A6", lw=3, alpha=0.6,
label="trip price guess")
plt.autoscale(tight=True)
p3 = plt.Rectangle((0, 0), 1, 1, fc=sp3.get_facecolor()[0])
plt.title("Prior distributions for unknowns: the total price, the snowblower's price, and the trip's price")
plt.legend([p3], [sp3.get_label()]);
plt.xlabel("Price");
plt.ylabel("Density")
import pymc as pm
data_mu = [3e3, 12e3]
data_std = [5e2, 3e3]
mu_prior = 35e3
std_prior = 75e2
true_price = pm.Normal("true_price", mu_prior, 1.0 / std_prior ** 2)
prize_1 = pm.Normal("first_prize", data_mu[0], 1.0 / data_std[0] ** 2)
prize_2 = pm.Normal("second_prize", data_mu[1], 1.0 / data_std[1] ** 2)
price_estimate = prize_1 + prize_2
@pm.potential
def error(true_price=true_price, price_estimate=price_estimate):
return pm.normal_like(true_price, price_estimate, 1 / (3e3) ** 2)
mcmc = pm.MCMC([true_price, prize_1, prize_2, price_estimate, error])
mcmc.sample(50000, 10000)
price_trace = mcmc.trace("true_price")[:]
____________________________________________________________________________
[Output]:
[-----------------100%-----------------] 50000 of 50000 complete in
10.9 sec
____________________________________________________________________________
figsize(12.5, 4)
import scipy.stats as stats
# Plot the prior distribution.
x = np.linspace(5000, 40000)
plt.plot(x, stats.norm.pdf(x, 35000, 7500), c="k", lw=2,
label="prior distribution\n of suite price")
# Plot the posterior distribution, represented by samples from the MCMC.
_hist = plt.hist(price_trace, bins=35, normed=True, histtype="stepfilled")
plt.title("Posterior of the true price estimate")
plt.vlines(mu_prior, 0, 1.1*np.max(_hist[0]), label="prior's mean",
linestyles="--")
plt.vlines(price_trace.mean(), 0, 1.1*np.max(_hist[0]), label="posterior's mean", linestyles="-.")
plt.legend(loc="upper left");
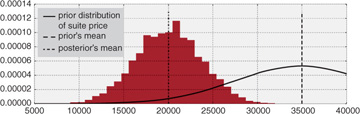
Figure 5.2.2: Posterior of the true price estimate
Notice that because of the snowblower prize and trip prize and subsequent guesses (including uncertainty about those guesses), we shifted our mean price estimate down about $15,000 from the previous mean price.
A frequentist, seeing the two prizes and having the same beliefs about their prices, would bid μ1 + μ2 = $35,000, regardless of any uncertainty. Meanwhile, the naive Bayesian would simply pick the mean of the posterior distribution. But we have more information about our eventual outcomes; we should incorporate this into our bid. We will use the loss function to find the best bid (best according to our loss).
What might a contestant’s loss function look like? I would think it would look something like:
def showcase_loss(guess, true_price, risk=80000): if true_price < guess: return risk elif abs(true_price - guess) <= 250: return -2 * np.abs(true_price) else: return np.abs(true_price - guess - 250)
where risk is a parameter that defines how bad it is if your guess is over the true price. I’ve arbitrarily picked 80,000. A lower risk means that you are more comfortable with the idea of going over. If we do bid under and the difference is less than $250, we receive both prizes (modeled here as receiving twice the original prize). Otherwise, when we bid under the true price, we want to be as close as possible, hence the else loss is a increasing function of the distance between the guess and true price.
For every possible bid, we calculate the expected loss associated with that bid. We vary the risk parameter to see how it affects our loss. The results are shown in Figure 5.2.3.
figsize(12.5, 7)
# NumPy-friendly showdown_loss
def showdown_loss(guess, true_price, risk=80000):
loss = np.zeros_like(true_price)
ix = true_price < guess
loss[~ix] = np.abs(guess - true_price[~ix])
close_mask = [abs(true_price - guess) <= 250]
loss[close_mask] = -2 * true_price[close_mask]
loss[ix] = risk
return loss
guesses = np.linspace(5000, 50000, 70)
risks = np.linspace(30000, 150000, 6)
expected_loss = lambda guess, risk: showdown_loss(guess, price_trace,
risk).mean()
for _p in risks:
results = [expected_loss (_g, _p) for _g in guesses]
plt.plot(guesses, results, label="%d"%_p)
plt.title("Expected loss of different guesses, \nvarious risk levels of \
overestimating")
plt.legend(loc="upper left", title="risk parameter")
plt.xlabel("Price bid")
plt.ylabel("Expected loss")
plt.xlim(5000, 30000);
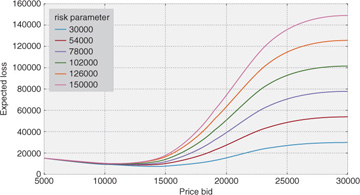
Figure 5.2.3: Expected loss of different guesses, various risk levels of overestimating
Minimizing Our Losses
It would be wise to choose the estimate that minimizes our expected loss. This corresponds to the minimum point on each of the curves on the previous figure. More formally, we would like to minimize our expected loss by finding the solution to
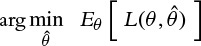
The minimum of the expected loss is called the Bayes action. We can solve for the Bayes action using SciPy’s optimization routines. The function in fmin in the scipy.optimize module uses an intelligent search to find a minimum (not necessarily a global minimum) of any univariate or multivariate function. For most purposes, fmin will provide you with a good answer.
We’ll compute the minimum loss for the Showcase example in Figure 5.2.4.
import scipy.optimize as sop
ax = plt.subplot(111)
for _p in risks:
_color = ax._get_lines.color_cycle.next()
_min_results = sop.fmin(expected_loss, 15000, args=(_p,),disp=False)
_results = [expected_loss(_g, _p) for _g in guesses]
plt.plot(guesses, _results, color=_color)
plt.scatter(_min_results, 0, s=60,
color=_color, label="%d"%_p)
plt.vlines(_min_results, 0, 120000, color=_color, linestyles="--")
print "minimum at risk %d: %.2f"%(_p, _min_results)
plt.title("Expected loss and Bayes actions of different guesses, \n \
various risk levels of overestimating")
plt.legend(loc="upper left", scatterpoints=1,
title="Bayes action at risk:")
plt.xlabel("Price guess")
plt.ylabel("Expected loss")
plt.xlim(7000, 30000)
plt.ylim(-1000, 80000);
____________________________________________________________________________
[Output]:
minimum at risk 30000: 14189.08
minimum at risk 54000: 13236.61
minimum at risk 78000: 12771.73
minimum at risk 102000: 11540.84
minimum at risk 126000: 11534.79
minimum at risk 150000: 11265.78
____________________________________________________________________________
____________________________________________________________________________
[Output]:
(-1000, 80000)
____________________________________________________________________________
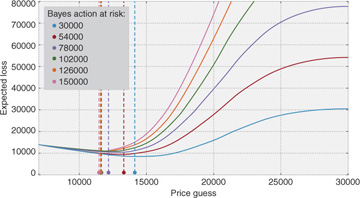
Figure 5.2.4: Expected loss and Bayes actions of different guesses, various risk levels of overestimating
As we decrease the risk threshold (care about overbidding less), we increase our bid, willing to edge closer to the true price. It is interesting how far away our optimized loss is from the posterior mean, which was about 20,000.
Suffice it to say, in higher dimensions, being able to eyeball the minimum expected loss is impossible. That is why we require use of SciPy’s fmin function.
Shortcuts
For some loss functions, the Bayes action is known in closed form. We list some of them here.
If using the mean-squared loss, the Bayes action is the mean of the posterior distribution; that is, the value
- Eθ[θ]
minimizes
. Computationally, this requires us to calculate the average of the posterior samples (see Chapter 4 on the Law of Large Numbers).
- Whereas the median of the posterior distribution minimizes the expected absolute loss, the sample median of the posterior samples is an appropriate and very accurate approximation to the true median.
- In fact, it is possible to show that the MAP estimate is the solution to using a loss function that shrinks to the zero-one loss.
Maybe it is clear now why the first-introduced loss functions are used most often in the mathematics of Bayesian inference: No complicated optimizations are necessary. Luckily, we have machines to do the complications for us.