- Overview
- Statistics and Machine Learning
- The Impact of Big Data
- Supervised and Unsupervised Learning
- Linear Models and Linear Regression
- Generalized Linear Models
- Generalized Additive Models
- Logistic Regression
- Enhanced Regression
- Survival Analysis
- Decision Tree Learning
- Bayesian Methods
- Neural Networks and Deep Learning
- Support Vector Machines
- Ensemble Learning
- Automated Learning
- Summary
Neural Networks and Deep Learning
Deep learning has recently received considerable attention in business media; analysts successfully used the technique in a number of highly visible data mining competitions. Deep Learning is an extension of Neural Networks; in this section, we discuss both techniques.
Neural Networks
Artificial neural networks are computational models inspired by the study of brains and the nervous system; they consist of a network of nodes (“neurons”) connected by directed graphs (“synapses”). Neuroscientists developed neural networks as a way to study learning; their methods are broadly applicable to problems in predictive analytics.
In a neural network, each neuron accepts mathematical input, processes the inputs with a transfer function, and produces mathematical output with an activation function. Neurons operate independently on their local data and on input from other neurons.
Neural networks may use a range of mathematical functions as activation functions. While a neural network may use linear functions, analysts rarely do so in practice; a neural network with linear activation functions and no hidden layer is a linear model. Analysts are much more likely to use nonlinear activation functions, such as the logistic function; if a linear function is sufficient to model the target, there is no reason to use a neural network.
The nodes of a neural network form layers, as shown in Exhibit 9.6. The input layer accepts mathematical input from outside the network, while the output layer accepts mathematical input from other neurons and transfers the results outside the network. A neural network may also have one or more hidden layers that process intermediate computations between the input layer and output layer.
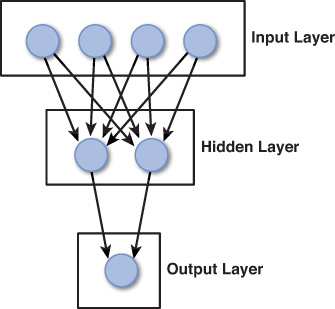
Exhibit 9.6 Neural Network Topology
When you use neural networks for predictive analytics, the first step is to specify the network topology. The predictor variables serve as the input layer, and the output layer is the response measure. The optional hidden layers enable the model to learn arbitrarily complex functions. Analysts use some heuristics to determine the number of hidden layers and their size, but some trial and error is required to determine the best network topology.
There are many different neural network architectures, distinguished by topology, flow of information, mathematical functions, and training methods. Widely used architectures include the following:
- Multilayer perceptron
- Radial basis function network
- Kohonen self-organizing network
- Recurrent networks (including Boltzmann machines)
Multilayer perceptrons, which are widely used in predictive analytics, are feedforward networks; this means that a neuron in one layer can accept input from any neuron in a previous layer but cannot accept input from neurons in the same layer or subsequent layers. In a multilayer perceptron, the parameters of the model include the weights assigned to each connection and to the activation functions in each neuron. After the analyst has specified a neural network’s topology, the next step is to determine the values for these parameters that minimize prediction errors, a process called training the model.
Many methods are available to train a neural network; for multilayer perceptrons, the most widely used class of methods is backpropagation, which uses a data set in which values of the target (output layer) are known to infer parameter values that minimize errors. The method proceeds iteratively; first computing the target value with training data and then using information about prediction errors to adjust weights in the network.
Several different backpropagation algorithms exist; gradient descent and stochastic gradient descent are the most widely used. Gradient descent uses arbitrary starting values for the model parameters and computes an error surface; it then seeks out a point on the error surface that minimizes prediction errors. Gradient descent evaluates all cases in the training data set each time it iterates; stochastic gradient descent works with a random sample of cases from the training data set. Consequently, stochastic gradient descent converges more quickly than gradient descent but may produce a less accurate model. The gradient descent algorithms can also train other types of models, including support vector machines and logistic regression.
Alternative algorithms for training a backpropagation neural network include the Broyden–Fletcher–Goldfarb–Shanno (BFGS) algorithm and its limited memory variant (L-BFGS) and the conjugate gradient algorithm. These algorithms can perform significantly better at minimizing prediction errors but tend to require more computing resources.
Radial basis function (RBF) networks have one or more hidden layers representing distance measures modeled with a Gaussian function. Analysts train RBF networks with a maximum likelihood algorithm. Compared to multilayer perceptrons, RBF networks are less likely to confuse a local minimum in the error surface for the desired global minimum; however, they are also more prone to overfitting.
Kohonen self-organizing networks (self-organizing maps) are a technique for unsupervised learning with limited application in predictive analytics. Refer to the appendix for a discussion of unsupervised learning with neural networks.
In a recurrent neural network (RNN), information flows in either direction among the layers; this contrasts with feedforward networks, where information flows in one direction only: from the input layer to the hidden layers to the output layer. The most important type of RNN is the restricted Boltzmann machine, an architecture used in deep learning (discussed in the following section).
The key strength of neural networks is their ability to model very complex nonlinear functions. Neural networks are also well suited to highly dimensional problems, where the number of potential predictors is very large.
The key weakness of neural networks is their tendency toward overlearning. A network learns to minimize prediction error on the training data, which is not the same thing as minimizing prediction error in a business application. As with other modeling techniques, analysts must test models produced with neural networks on an independent sample.
Analysts using the neural network technique must make a number of choices about the network topology, transfer functions, activation functions, and the training algorithm. Because there is very little theory to guide the choices, the analyst must rely on trial and error to find the best model. Consequently, neural networks tend to consume more analyst time to produce a useful model.
Leading commercial software packages for machine learning, including IBM SPSS Modeler, RapidMiner, SAS Enterprise Miner, and Statistica, support neural networks, as do in-database libraries such as dbLytix and Oracle Data Mining. Multiple packages in open source R support neural networks; in Python, the PyBrain package offers extensive capabilities.
Deep Learning
Deep learning is a class of model training techniques based on feature learning, or the capability to learn a concise set of “features” from complex unlabeled data. In practice, a deep neural network is a neural network with multiple hidden layers trained sequentially with unsupervised learning techniques.
Interest in deep learning stems from a number of notable recent successes in machine learning competitions:
- International Conference on Document Analysis and Recognition (2009)
- IJCNN Traffic Sign Recognition Competition (2011, 2012)
- ISBI Segmentation of Neuronal Structures in Electron Microscopy (2012)
- Merck Molecular Activity Challenge (2012)
The theory of deep learning dates to the 1980s; however, practical application lagged due to the computational complexity and resources needed. The increased availability and reduced cost of GPU devices and other platforms for high-performance computing has provided analysts with the computing power to experiment with deep learning techniques.
Deep neural networks are prone to overfitting due to the introduction of additional abstraction layers; analysts manage this tendency with regularization techniques. Models must be tested and validated to ensure they generalize to fresh cases.
Commercial software for deep learning is limited at present. Neither SAS nor SPSS currently support the capability out of the box: PROC Neural in SAS Enterprise Miner 13.1 permits users to build neural networks with an unlimited number of hidden layers but lacks the ability to build Boltzmann machines, a necessary tool for deep learning. There are, however, a number of open source deep learning libraries available in C, Java, and Python as well as a MATLAB Toolbox.