Microsoft SQL Server Analysis Services: Introduction to OLAP and Its Role in Business Intelligence
In the past decade, Microsoft SQL Server Analysis Services established itself as one of the leaders in the Business Intelligences systems market. Analysis Services helps managers, employees, customers, and partners to make more informed business decisions by enabling them to analyze information accumulated during a company's day-to-day operations.
Success of Analysis Services and the entire Business Intelligence market was predefined by incredible growth of amounts of data accumulated as a result of everyday functioning of a large number of companies. Today it's hard to imagine a business or an organization that doesn't use an online transaction processing (OLTP) system. OLTP systems provide means to highly efficient execution of a large number of small transactions and reliable access to data stored in the result of the transactions.
The volume of the data stored and processed for one day by an OLTP system could be several gigabytes per day; after a period of time, the total volume of data can reach to the tens and even hundreds of terabytes. Such a large volume of data can be hard to store, but it is a valuable source of information for understanding the way the enterprise functions. This data can prove very helpful for making projections that lead to successful strategic decisions, and for improving everyday decision making.
It's easy to see why analysis of data has become so important to the management of modern enterprises. However, OLTP systems are not well suited to analyzing data. In the past decades, an entire new market has emerged for systems that can provide reliable and fast access for analyzing very large amounts of data: online analytical processing (OLAP). OLAP enables managers, executives, and analysts to gain insight into data using fast, interactive, and consistent interfaces to a wide variety of possible views of information. For example, with OLAP solution, you can request information about company sales in Europe over the year, then drill down to the sales of computers in September, calculate year-to-date sales or compare revenue figures with those for the same products sold in January, and then see a comparison of TV sets sales in Europe in the same time period.
Because OLAP systems are designed specifically for analysis, they typically don't need to both read and write data. All that is necessary for analysis is reading data. With this emphasis on reading only, OLAP systems enjoy a speed advantage over their OLTP cousins. However, a read-only approach to the database architecture is not the only distinction of the OLAP solution. The following rules distinguish OLAP systems from relational databases:
-
Multidimensional data structures
OLAP solutions typically use multidimensional data structures that allow analysts and managers to analyze numeric values from different perspectives, such as time, customers, products, and others.
-
Consistently fast data access
Architecture of the system allows constantly fast access to the data. To ensure fast, predictable query times, OLAP solutions typically pre-aggregate data.
-
Intuitive interface
Skilled analysts and nontechnical users alike can manipulate and analyze data; they can generate reports without involving their organization's IT department.
-
Complex calculations
With multiple dimensions come more complex, cross-dimensional calculations. You might need to calculate the subtotal of sales for the state Washington, for example, to be expressed as a percentage of the whole U.S. sales. Further, this result may be presented as part of a time-series analysis (for instance, current month versus last month, versus a year ago).
The Multidimensional Data Model
The design and development of the multidimensional database—especially Microsoft SQL Server Analysis Services, the system designed and developed by the authors of this book—was inspired by the success of relational databases. If you're already familiar with relational databases, you'll recognize some of the terminology and architecture. But, to understand Analysis Services, you must first understand multidimensional data models, how this model defines the data and processes it, and how the system interacts with other data storing systems, primarily with the relational data model.
The multidimensional data model for Analysis Services consists of three more specific models:
- The conceptual data model
- The application data model
- The physical data model
The Conceptual Data Model
The conceptual data model contains information about how the data is represented and the methods for defining that data. It defines data in terms of the tasks that the business wants to accomplish using the multidimensional database. To define conceptual data model, you use the user specifications for the structure and organization of the data, rules about accessing the data (that is, security rules), and calculation and transformation methods.
In a sense, the conceptual data model serves as a bridge between a business model and the multidimensional data model. The solutions architect is the primary user for the conceptual data model. We use Data Definition Language (DDL) and MDX (Multidimensional Extensions) script for the creation of the conceptual model. You can also use Business Intelligence Development Studio to develop the conceptual data model.
The Application Data Model
The application model defines the data in a format that can be used by the analytical applications that will present data to a user in a way that he can understand and use. The primary user for the application data model is the client application, which exposes the model to the user. The application model is built with the MDX language and XML for Analysis protocol. The chapters of Part 3, "Using MDX to Analyze Data," contain detailed information about MDX and a few of most commonly used client applications. The chapters of Part 7, "Accessing Data in Analysis Services," contain information about protocol used by Analysis Services to communicate with client applications.
The Physical Data Model
As in the arena of relational databases, the physical model defines how the data is stored in physical media:
- Where it is stored—What drive (or maybe on the network), what types of files the data is stored in, and so on
- How it is stored—Compressed or not, how it's indexed, and so on
- How the data can be accessed—Whether it can be cached, where it can be cached, how it is moved into memory, and so on
The database administrator is the primary user for the physical data model. We use XML-based commands for manipulation of data on the physical layer.
Figure 1.1 shows relationships between three parts of multidimensional model.
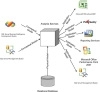
Figure 1.1 Submodels of the multidimensional model.
You use SQL Server Business Intelligence Development Studio or SQL Server Management Studio to define a conceptual data model, also known as a Unified Dimensional Model (UDM) or cube. After the conceptual model is defined, you populate it with data by loading/processing the data from the relational database. At this time, you define the physical data model—partitioning scheme of the data, indexing scheme, and so on. The application model of Analysis Services consists of standard data access interfaces. Client applications use those interfaces: XML for Analysis and MDX to communicate with Analysis Services. More than hundred applications available today support the application model of Analysis Services and can work with any Analysis Services cubes.
Unified Dimensional Model
The UDM of Microsoft SQL Server Analysis Services makes it possible for you to set up your system so that different types of client applications can access data from both the relational and the multidimensional databases in your data warehouse, without using separate models for each.
It's been a common industry practice for some time now to build data warehouses that include a relational database for storing data and a multidimensional database for analyzing data. This practice developed because the large volumes of data that multidimensional databases were developed to analyze are typically stored in relational databases. The data would be moved to the multidimensional database for analysis, but relational database would continue to serve as primary storage.
Therefore, it makes sense that the interaction between the stored data and the multidimensional database where it can be analyzed has been an important component of multidimensional database architecture. Our goal for Analysis Services, put simply, is speedy analysis of the most up-to-date data possible.
The speedy and up-to-date parts are what present the challenge. The data in OLTP systems is constantly being updated. But we wouldn't want to pour data directly from an OLTP system into a multidimensional database, because OLTP data is easily polluted by incomplete transactions or incomplete data entered in a transaction. In addition, you don't want your analysis engine to access the OLTP data directly, because that could disrupt work and reduce productivity.
In a data warehouse, OLTP data is typically transformed and stored in a relational database and then loaded into a multidimensional database for analysis. To connect the two databases, you can choose from three methods, each one using a different kind of interaction:
- Relational OLAP (ROLAP), in which no data is stored directly in the multidimensional database. It is loaded from the relational database when it is needed.
- Multidimensional OLAP (MOLAP), in which data is loaded into the multidimensional database and cached there. Future queries are run against the cached data.
- Hybrid OLAP (HOLAP), in which the aggregated data is cached in the multidimensional database. When the need arises for more detailed information, that data is loaded from the relational database.
In earlier versions of Analysis Services, the multidimensional part of the data warehouse was a passive consumer of data from the relational database. The functions of storing data and analyzing data were not only separate, but you had to understand two models—one for accessing a relational database and one for accessing a multidimensional database.
Some client applications would use one model, and others would use the other model. For example, reporting applications traditionally would access the data in a relational database. On the other hand, an analysis application that has to look at the data in many different ways would probably access the data in the multidimensional database, which is designed specifically for that sort of use.
Now, the UDM offers a substantially redefined structure and architecture so that the one model (UDM) serves the purposes of any client application. You no longer have to understand two models; we're providing a unified model. Figure 1.2 shows how many different client applications can use UDM to access data in a variety of different data stores.

Figure 1.2 The UDM provides a unified model for accessing and loading data from varied data sources.
Analysis Services uses proactive caching to ensure that the user of the client application is always working with predictable data latency. In essence, proactive caching is a mechanism by which the user can schedule switching from one connection mode (ROLAP, MOLAP, or HOLAP) to another. For example, the user might set his system to switch from MOLAP to ROLAP if the data in the MOLAP system is older than, say, four hours.
With UDM at the center of the multidimensional model, you no longer need to have different methods of data access for different data sources. Before UDM, every system had a number of specialized data stores, each one containing data that was stored there for a limited number of users. Each of these data sources would likely require specific methods of data access for loading data into the multidimensional model. With Analysis Services, all the data of the enterprise is available through the UDM, even if those data sources are located on different types of hardware running different operating systems or different database systems. OLAP now serves as an intermediate system to guarantee effective access to the data.
Basic Concepts
When you start to build a multidimensional model, you think about business entities your organization operates with and about values that you need to analyze. For example, in our fictional organization—a chain of grocery stores known as Food Mart—we operate with warehouses, stores, products, customers, and different currencies, as shown in Figure 1.3. Those business entities became dimensions of our multidimensional model. Typically, you want to analyze data in a context of a time periods, and therefore the Time dimension is present in almost all multidimensional models. Actual values or facts that you are analyzing, such as sales, costs, and units, are called measures.

Figure 1.3 A multidimensional model consists of dimensions and measures.
Each individual element of the dimension is called a member. For example, "Club 1% Milk" is a member of the Products dimension, Irina Gorbach is a member of the Customers dimension, and January 1997 is a member of the Time dimension.
Each business entity usually has multiple characteristics. For instance, a customer can have the following properties: name, gender, city, state, and country. You might look at the products by name, Stock Keeping Unit (SKU), brand, product family, product category, and so on. We call these characteristics of the business entity dimension attributes. Figure 1.4 shows dimension attributes.

Figure 1.4 Each dimension is defined by its attributes.
Dimension attributes are not completely independent from each other. For example, Year contains Quarter, and Quarter contains Month. We can say that Year, Quarter, and Month attributes are related to each other.
If members of different attributes have a hierarchical structure, attributes can be organized in a hierarchy. For example, you can create the hierarchy Calendar—Year > Quarter > Month within the Time dimension, because the year contains quarters and quarters contains months.
After data is loaded in the cube, you can access it with many client applications. Microsoft Excel is one of the most frequently used application. Figure 1.5 shows Excel 2007 exposing data stored in Analysis Services cube.
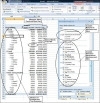
Figure 1.5 Accessing data in FoodMart 2008 sample using Excel 2007.
This Excel spreadsheet demonstrates sales and cost for products in different time periods based on the data stored in the FoodMart 2008 database.
In Chapter 2, "Multidimensional Space," we explain the terms that we use to describe multidimensional space.