- Current State of Data Quality
- Recognizing Dirty Data
- Data Quality Rules
- Data Quality Improvement Practices
- Enterprise-Wide Data Quality Disciplines
- Enterprise Architecture
- Business Sponsorship
- Conclusion
- References
Enterprise-Wide Data Quality Disciplines
Organizations have a number of data quality disciplines at their disposal, but rarely will they implement all disciplines at once because improving data quality is a process and not an event. This process is measured on a data quality maturity scale of 1–5. Depending on how fast an organization advances through the data quality maturity levels, it will either institute stringent, light, or no disciplines.
Data Quality Maturity Levels
An easy way to determine your organization’s level of data quality maturity is to look at your current data quality improvement activities. Figure 3.1 shows the common data quality improvement activities in each of the five data quality maturity levels based on Larry English’s adaptation of the capability maturity model (CMM) to data quality. The five levels are:
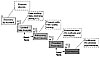
Figure 3.1 Data Quality Improvement Activities
Level 1: Uncertainty—At Level 1, the organization is stumbling over data defects as its programs abend (crash) or its information consumers complain. There is no proactive data quality improvement process, no data quality group, and no funding. The organization denies any serious data quality problems and considers data analysis a waste of time. Or the CIO is ready to retire and doesn’t want anything to disrupt it. Basically, the organization is asleep and doesn’t want to be awakened.
Level 2: Awakening—At Level 2, the organization performs some limited data analysis and data correction activities, such as data profiling and data cleansing. There still is no enterprise-wide support for data quality improvement, no data quality group, and no funding. However, a few isolated individuals acknowledge their dirty data and want to incorporate data quality disciplines in their projects. These individuals can be data administrators, database administrators, developers, or business people.
Level 3: Enlightenment—At Level 3, the organization starts to address the root causes of its dirty data through program edits and data quality training. A data quality group is created and funding for data quality improvement projects is available. The data quality group immediately performs an enterprise-wide data quality assessment of their critical files and databases, and prioritizes the data quality improvement activities. This group also institutes several data quality disciplines and launches a comprehensive data quality training program across the organization.
Level 4: Wisdom—At Level 4, the organization proactively works on preventing future data defects by adding more data quality disciplines to its data quality improvement program. Managers across the organization accept personal responsibility for data quality. The data quality group has been moved under a chief officer—either the CIO, COO, CFO, or a new position, such as a chief knowledge officer (CKO). Metrics are in place to measure the number of data defects produced by staff, and these metrics are considered in the staff’s job performance appraisals. Incentives for improving data quality have replaced incentives for cranking out systems at the speed of light.
Level 5: Certainty—At Level 5, the organization is in an optimization cycle by continuously monitoring and improving its data defect prevention processes. Data quality is an integral part of all business processes. Every job description requires attention to data quality, reporting of data defects, determining the root causes, improving the affected data quality processes to eliminate the root causes, and monitoring the effects of the improvement. Basically, the culture of the organization has changed.
Standards and Guidelines
Data quality does not happen by accident. Organizations must establish standards and guidelines for all personnel to follow to ensure that data quality is addressed during the entire lifecycle of a system. For example, standards should be established for defining the data, naming the data, establishing domains and business rules, and modeling the data. Guidelines should be in place for data entry, edit checking, validating and auditing of data, correcting data errors, and removing the root causes of data contamination. Training and familiarization with the standards and guidelines should be required of all data entry staff, developers, data stewards, and information consumers.
Standards and guidelines should also include policies and procedures, such as operating procedures, change-control procedures, issue management procedures, and data dispute resolution procedures. Additional policies and procedures should be considered for the communication processes, estimating guidelines, roles and responsibilities, and standard documentation formats.
Development Methodology
A development methodology is a common roadmap that provides a complete list of all the major activities and tasks to be performed on projects. The trouble with traditional methodologies is that they do not support cross-organizational data integration activities because operational systems were rarely designed with integration in mind. But increasing demand for integrated systems (including ERP, CRM, and DW) requires a new type of data-driven methodology that includes the appropriate data quality improvement tasks. For example, the methodology must have a separate development step for incrementally building the enterprise logical data model and enforcing data standardization across all projects.
Data Naming and Abbreviations
Data naming and abbreviation standards provide consistency and a common look and feel that are useful for both developers and business people. Proven standards can be applied, such as the convention of name compositions using prime words, qualifiers or modifiers, and class words. Data administrators are usually trained in the various industry-standard naming conventions.
Abbreviations are part of naming standards, but they apply only to physical names, such as column names, table names, or program names. Business names should always be spelled out for clarity and understanding regardless of how long they are. You should publish a standard enterprise-wide abbreviations list that includes industry-specific and organization-specific acronyms. Every project team should use these abbreviations and acronyms.
Metadata
Metadata is descriptive contextual information about architectural components. Metadata can be business metadata, technical metadata, process metadata, and usage metadata. Large amounts of business metadata can be collected about business functions, business processes, business entities, business attributes (data elements), business rules, and data quality. Technical metadata represents the physical architectural components, such as programs, scripts, databases, tables, columns, keys, and indices. Process metadata describes any type of program logic that manipulates data during data capture, data movement, or data retrieval. Usage metadata is statistical information about how systems are used by the business people. For example, what type of data is accessed, by whom, how often, and for what purpose.
You should set up standards or guidelines that govern who captures which metadata components and how, when, and where to capture them. The metadata repository should be set up in such a way that it supports the standards for metadata capture and usage. Metadata is discussed in more detail in Chapter 4, "Metadata."
Data Modeling
There is a difference between logical data modeling and physical data modeling. A logical data model is a normalized business model and a physical data model is a denormalized database design model, also known as a logical database design. These two different types of data models are described in Chapter 5, "Data Modeling." Data quality must be addressed in both sets of models. In addition, the data models themselves must meet data-modeling quality standards with respect to data policies and modeling rules, such as compliance with naming conventions, consistent use of data types and data domains for semantically equivalent attributes, and so on.
For the purpose of finding redundant and inconsistent data, logical entity-relationship modeling with complete data normalization is still the most effective technique because it is a business analysis technique that includes identification, rationalization, and standardization of data through business metadata. Because every business activity or business function uses or manipulates data in some fashion, a logical data model documents those logical data relationships and the business rules, regardless of how the data or the functions are implemented in the physical databases and applications.
Logical data models created for individual applications should be merged into one cohesive, integrated enterprise logical data model. This activity is usually performed by the data administration department, which might be part of the data quality group. The enterprise logical data model is the baseline business information architecture into which physical files and databases are mapped. You should establish standards for creating logical data models as part of system development activities and for merging the models into the enterprise logical data model.
Data Quality
Because most organizations have a lot of dirty data—too much to cleanse it all—they must establish guidelines about triaging (categorizing and prioritizing) dirty data for cleansing. Some data is critical to the organization, some is important but not critical, and some is nice to have but relatively insignificant to the business people. You should create standards that define acceptable data quality thresholds for each of these categories and specify how to measure data quality during and after database updates. Processing rules for error handling and suspending dirty data records for subsequent correction also should be part of the standards.
Testing
You should specify what types of testing should be performed during system development and who should participate in the various types of testing. Specific types of testing include unit testing, integration or regression testing, performance testing, quality assurance testing, and user acceptance testing. Guidelines should be established that describe the types of test cases required, how much regression testing to perform, and under what circumstances to regression test. Testing guidelines should include a brief description of a test plan, perhaps even a template, as well as instructions for how to organize and manage the various testing activities.
Reconciliation
Similar to testing, yet in a separate category, is reconciling the results of any data manipulation, which is the process of capturing, storing, extracting, merging, separating, copying, moving, changing, or deleting data. This is especially true for DW applications that extract data from multiple operational source files and merge the data into one target database. If your organization has adopted an architected data mart strategy, then the various data marts also have to be reconciled to each other to guarantee consistency. This includes having one central staging area with extensive reconciliation programming for every input-process-output module.
Security
Security guidelines apply to operational systems as well as decision-support systems. The only time data security can be slightly relaxed is in data marts where data is highly summarized and the ability to drill down to the details is not enabled. You should establish security standards to guide the project teams on what types of security measures are mandatory for what types of data exposure. The security standards should have guidelines for categorizing data sensitivity and risks of exposure for the organization. Security standards should cover application security, network security, database security, and Web security against intrusions, hackers, and viruses.
Data Quality Metrics
Data quality metrics ordinarily reflect the explicit as well as the implicit business principles of an organization. Business principles are explicit if stated in mission or vision statements, implicit if they are just "understood" by the staff. For example, if an organization rewards project managers for meeting deadlines even though their applications are full of errors, while it punishes project managers for missing deadlines even though their applications are flawless, then the implicit principle is "speed before quality." Therefore, when creating data quality metrics, the explicit as well as implicit business principles must be reviewed and changed, if necessary, to support the metrics.
Another important aspect to measuring data quality is setting goals. Organizations need to be clear on where they are today and what they’re trying to achieve in the short term, medium term, and long term. What are the priorities in the organization? Should operational data be addressed or only analytical data? Should financial data be cleansed first or a specific subject area for an application, such as CRM? What is the plan for incrementally managing data quality improvements? What are the staffing requirements and what are the roles and responsibilities for a data quality improvement initiative? These questions must be answered to develop meaningful and actionable data quality metrics.