Social Media Analytics: Whose Comments Are We Interested In?
- Looking for the Right Subset of People
- Are They Happy or Unhappy?
- Location and Language
- Age and Gender
- Eminence, Prestige, or Popularity
- Summary
- All opinions are not equal. Some are a very great deal more robust, sophisticated, and well supported in logic and argument than others.
- —Douglas Adams, The Salmon of Doubt 1
Up to this point, we have concerned ourselves with what data to analyze while ensuring that what we selected is germane to our topic. In this chapter, we explore how important it is to determine whose comments we are interested in. A few examples are as follows:
- If we are interested in getting objective feedback on a product from a specific company, we might want to make sure that we can identify or exclude this company’s employees from the pool of content under analysis.
- Similarly, we need to ask: Are we interested in comments from the general public, or are we interested in the comments of C-level employees (that is, chief marketing officers or chief information officers)?
- Also, are we interested only in people who have a positive bias toward a company or those with a strong negative bias?
Looking for the Right Subset of People
At the beginning of a social analytics project, analysts spend a fair amount of time thinking about the ultimate goals of the project and the results that we expect to get at the conclusion of the project. This upfront analysis will go a long way in determining the appropriate target segment of the analysis.
During the definition of a typical social media analysis project, requesters will (or should) explicitly point out the “who” (whose opinion are they interested in?) or will give the researcher or the model builder sufficient hints or guidance. Various attributes can be used to segment or target the audience that we’re interested in. Some of them are described in the following sections.
Employment
Do we want the opinions of employees or nonemployees?
For example, if a company launches a new product or service and wants to see how the marketplace is reacting to that product or service in social media, it might prefer to exclude the comments of its own employees. In other situations, we might exclusively focus on the employee population if the intent is to learn how they are responding to a new product, service, or strategy. In a project that we worked on, IBM was interested in learning about the marketplace reaction of a brand-new product type. The marketing team specifically asked us to exclude the comments and sentiments of IBMers to understand sentiment from “neutral” people so as not to bias the results.
Sentiment
Are we looking for comments from people with a positive bias or negative bias?
For example, if the object of social media analysis is to detect customer support issues, it makes sense to focus only on posts with a clear negative bias. You might argue that highlighting positive customer experiences is just as important and probably needs to be considered as well. Another common use case involves trying to compare the sentiment about a variety of products that a company is providing to the marketplace. In this situation, we may consider opinions from all ranges of demographics and keep score about the number of positive, negative, or neutral comments. Sometimes, the purpose of a project is merely to find how many people or comments mention the company’s product versus a competitor’s product. In this case, we may (initially) ignore sentiment and consider all comments without exclusions.
A few years ago, there was a civil movement called Occupy Wall Street in the United States. Numerous people congregated around specific commercial buildings to express their silent protests against what they believed to be unfair practices. During this time, as a validation of some of our analytics capabilities, we built an experimental social listening model to detect whether there was any impact to an IBM location where some key customer meetings were being conducted. In this case, we built a model that focused on snippets of information that may have negative sentiment about IBM and then specifically looked for any mentions of protests or civil actions.
In many cases, sentiment is a result of an analysis phase. However, in some instances, the scope and nature of the project determine whether we should include comments only from people who have either a favorable view or an unfavorable view of our topic. In cases like these, we are able to take this information into account in the very initial phase of the project and focus only on a specific subset of people.
Location or Geography
Do we want to focus on comments from people who live in a specific location?
One of the projects that we were involved in dealt with issues around water in South Africa. In this particular project, we were clearly interested in comments from people in South Africa about the variety of issues and questions around the current and future needs and use of clean and healthy water. Sometimes we may be interested in comments from all over the world, but valuable insights can emerge when we classify the analytics by region.
Language
Is the language of the content important to us?
Some projects require us to understand what is specifically being said about a company’s product or service in a particular local language. For example, if a company wants to do some market research around the market’s appetite for a machine translation tool in Spanish-speaking countries, it will be interested in content contributed by individuals in the Spanish language.
Age
Is the age of content author important to the project at hand?
There is a lot of discussion in popular media about the work habits of Generation Xers. Those in Generation X (or Gen X) were born after the Western Post–World War II baby boom. As a point of reference, most consider those with birth dates ranging from the early 1960s to the early 1980s as being part of this demographic. If a company’s Human Resources department wanted to study the experience of its newly hired Gen Xers, we would have to determine a way to segment the population based on age.
Gender
Are we specifically interested in comments of men or women?
Gender also becomes an important attribute upon which we may segment audience for a particular project. If an organization is creating training and educational materials to encourage more women to pursue higher studies in science- and mathematics-related disciplines, it may choose to focus exclusively on comments and feedback from women. Similarly, if a health-care company is undertaking research about male-pattern baldness, it would be served well by segmenting its audience to include only men.
In one case, we were asked to evaluate the comments that were made in social media during the introduction of a new movie trailer. Our client was interested not only in the reaction to the trailer, and by association the movie itself, but also if certain themes resonated with either males, females, or both. Again, the goal was to determine not only likeability of the movie, but also keys in how to market it.
Profession/Expertise
Do we need opinions from anybody in general, or do we need opinions from people who are working in a specific profession (such as the IT profession) in a specific industry (such as automotive)?
For example, if IBM is interested in learning about the reaction to the cognitive computing capabilities of IBM Watson in the area of health care, it is probably interested in the opinions of corporate users as opposed to home users.
Eminence or Popularity
Are we interested in opinions only from people of certain standing in the domain of the topic area?
A major aspect of a social media campaign for companies involves identifying who might be an “influencer” in a particular topic area or industry. For performing this type of analysis, we tend to spend a lot of time in developing rules to ensure we are able to narrow the solution space to identify a small subset of individuals that a company should target its marketing messages to.
Role
When dealing with social media analysis within a company’s intranet, are we interested in segmenting based on a specific job role?
For example, we are working on a project that computes a social scorecard for employees based on their participation in social media. There are some roles in which the job demands a lot of collaboration in social media, and then there are some people who might be working on highly specialized or highly sensitive projects in which they may not be allowed to share information in social media. Here, the type of role is very important in interpreting scores.
Specific People or Groups
Are we really interested in narrowing down our analysis to comments about or comments from a specific individual or a specific set of individuals?
A couple of years ago, we were asked to build an application to capture and display sentiment in near real time about tennis players participating in the US Open. In this case, we used names of players, their nicknames, and a variety of other aliases to ensure we were targeting the right segment. In another example, we were asked to identify how people in social media were reacting to a Lance Armstrong interview with Oprah Winfrey.
Do We Really Want ALL the Comments?
In Chapter 1, we discussed the concept of bias—or the skewing of a dataset based on a potentially inappropriate set of authors. Perhaps inappropriate is too strong of a word, but in some cases you might want to exclude the comments of your company’s employees. At IBM, we tend to look at ourselves as one of the best customers of our products and services, but sometimes IBMers are also among our most vocal critics. If we are looking to understand the true concerns or thoughts of our external customers and clients, we may want to exclude the subset of IBMers from the conversation. This is an example of the employment attribute that we discussed previously. Again, the purpose isn’t to exclude because these comments aren’t valuable, but in the spirit of openness and true sentiment or feelings, it may be useful to separate the comments.
In one example, we were asked to look at the social media activity around a new product launch. The client’s concern was that while there was a tremendous amount of money and time being invested in the various marketing campaigns, the sales hadn’t picked up as much as had been anticipated. A quick analysis of the discussion around the topic showed the level of activity over a four-week period (see Figure 3.1).
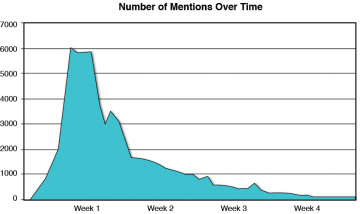
Figure 3.1 Social media remarks during an initial product announcement.
This graph shows the number of mentions of the particular product over time. It’s rather clear from this simple graphic that in the beginning, there was quite a bit of hype or discussion around this product launch, but over a short period of time, the discussion continued to decline almost to zero mentions.
What was even more disturbing about this analysis was who was having the conversations. We quickly looked at the top contributors to this thread of conversation and turned up the list shown in Figure 3.2.
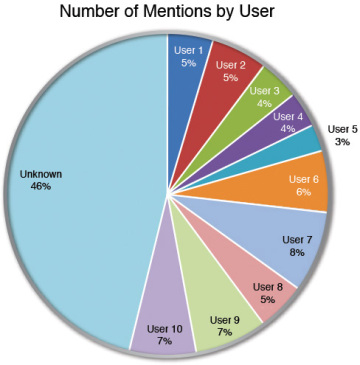
Figure 3.2 Top contributors to social media remarks during an initial product announcement.
A manual lookup of the top 10 users in this conversation revealed that at least 9 of them were employees of the company and represented nearly half the conversation (47%).
The conclusion we drew was that in the various social media and news venues, the employees were chatting about the new release, but given the slope of the curve in Figure 3.1, that conversation didn’t sustain itself. After the employees stopped talking, there was virtually no conversation. Clearly, a new marketing plan was needed since what was being said wasn’t being repeated, commented on, or perhaps even resonating with the public.