Holt-Winters Models
When you add a trend to a seasonal time series, you enter the bailiwick of what’s usually known as the Holt-Winters model. At first glance it might seem as though all you need to do is add a smoothing constant for the trend, as discussed in “Using Holt’s Linear Exponential Smoothing” in Chapter 3. And that first glance is largely correct—it’s only a little more complicated than that.
The smoothing form (and therefore the error correction form) of the equation to update each season is the same as is given earlier in this chapter, in “About the Season Smoothing Formulas.” Here it is again:

In words, the seasonal index for time t starts with Delta times the difference between the current observation and the current level of the series. To that is added (1 – Delta) times the index for the season associated with the current period, or the index that’s m seasons back.
No difference with the untrended seasonal series so far, but here’s the smoothing formula for the trend:
bt = γ(Lt - Lt-1)+(1-γ)bt-1
The difference between the current level, Lt, and the prior level, Lt–1, is taken to be the value of the current trend. That difference is multiplied by Gamma. Then we obtain the value of the immediately previous trend, bt-1, and multiply by (1 – Gamma). The sum of the two terms gets us a measure of the current trend.
Finally, the formula for the level is nearly the same as in the untrended seasonal model. Here’s the formula for a trended, seasonal model:
For the untrended, seasonal model the formula is this:

The difference between the two formulas is of course due to the presence of the bt-1 term in the former formula. The value of the trend as of time t – 1 is included because we’re now dealing with a trended series. In an untrended series we assume that there is no trend, that any difference between observations not caused by season or level is part of the error in the model.
But in a trended series the trend term is explicitly acknowledged. Let’s see how it works out in practice. Figure 5.26 has the first few observations from the well-known Series G, found in Box and Jenkins’s seminal book on ARIMA analysis. Series G is a count of airline passengers during each month of a 12-year period. Because the number of airline passengers is seasonal and, at the time (1949 through 1960), was trending strongly up, a Holt-Winters model is apt.
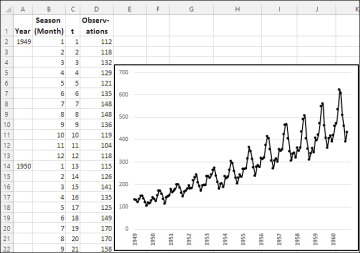
Figure 5.26 This time series clearly demonstrates both trend and seasonality.
The degree of seasonality appears to vary with the level of the series—that is, the higher the level of the series, the greater the swings between a year’s high month and its low month. This is a characteristic that suggests using a multiplicative model, which sounds a lot more forbidding than it really is, and which I discuss in Chapter 7, “Multiplicative and Damped Trend Models.” But for the time being, this chapter builds an additive Holt-Winters model that’s directly analogous to the one discussed in the preceding section, “Simple Seasonal Exponential Smoothing.”
Initializing the Forecasts
As Chapter 4 shows, there are many ways to go about initializing forecasts, and the more complicated the model the more choices you have. For example, in various sources, I’ve seen the Box-Jenkins Series G initialized using these approaches, among others, for a Holt-Winters analysis:
- By treating the mean of the first 12 months as the level of Period 0.
- By treating the difference between the result of Period 1 and each monthly observation during the first year as initial values of the seasonal indexes.
- By dividing the difference between the series’ 12th and 1st observations by 11; more generally, subtract the first observation from the mth observation and divide by (m – 1). Treat the result as the initial value of the monthly trend.
That’s a kind of rough-and-ready way to initialize the estimates of level, season, and trend; it might be a little crude compared to the more sophisticated approaches such as optimization, backcasting, and regression. But it’s quick, reasonable, and unlikely to steer you far wrong, particularly if you’re fortunate enough to have a lengthy baseline.
There’s also the issue of when you start your forecasts. Figure 5.27 shows the first 14 periods of the Box-Jenkins Series G data, with the actual observations in column D. (The full set of 144 observations is included in the workbook for this chapter, which you can download from the publisher’s website.) In Figure 5.27, the forecasts begin after the first year.
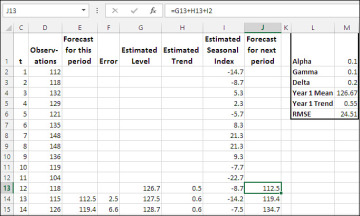
Figure 5.27 The forecasts, and therefore the forecast errors, start one year later in Figure 5.28 than they do in this figure.
Here’s how the formulas are set up in Figure 5.27:
The mean of the observations for the first encompassing period—in this case, that’s 1949—is entered with this formula in cell M4:
=AVERAGE(D2:D13)
The cell is named Year_1_Mean.
An estimate of the monthly trend during the first year is entered with this formula in cell M5:
=(D13-D2)/11
The cell is named Year_1_Trend. Bear in mind that it estimates the monthly trend during the first year.
The seasonal indexes for the first year are initiated with this formula in cell I2:
=D2-Year_1_Mean
The formula is simply the difference between the observation for the first month, less the mean observation for the first year. That formula is copied and pasted down through I13, to initialize each of the 12 seasonal estimates.
Cell G13 carries the first year’s mean into the column for level estimates with this formula:
=Year_1_Mean
Cell H13 carries the first year’s monthly trend into the column for trend estimates with this formula:
=Year_1_Trend
Cell J13 calculates the first smoothing forecast, this one for the 13th period, the first month of the second year. As with all the remaining forecasts, the one in cell J13 totals the level, the trend, and the seasonal index. This is the formula in cell J13:
=G13+H13+I2
Notice that the formula uses the seasonal value from the first month of the first year in cell I2.
The forecast for Period 13 is repeated from cell J13 to cell E14 with this formula in E14:
=J13
And the forecast error, the difference between the current observation and the forecast for the 13th period, is entered in cell F14 with this formula:
=D14-E14
The smoothing forecasts begin with the level estimate in cell G14, with this formula:
=Alpha*(D14-I2)+(1-Alpha)*(G13+H13)
You remove the seasonal effect from the observed value for the current period by subtracting the seasonal index (cell I2) from the observed value (cell D4); the result is multiplied by Alpha. The projected level (cell G13) and the projected trend (cell H13) as of the prior period are summed and the result is multiplied by (1 – Alpha). The two terms are summed to return the estimated series level as of Period 13.
The trend as of Period 13 is forecast in cell H14 with this formula:
=Gamma*(G14-G13)+(1-Gamma)*H13
The difference between the prior level and the current level (cells G13 and G14) is taken to be the current trend observation, and is multiplied by Gamma. The value in cell H13 is the forecast trend as of the prior period, and is multiplied by (1 – Gamma). The two terms are summed to return the estimated series trend as of Period 13.
The seasonal index as of Period 13 is forecast in cell I14 with this formula:
=Delta*(D14-G14)+(1-Delta)*I2
The difference between the current observation in cell D14 and the current level in cell G14 is taken to be the current seasonal effect; it is multiplied by Delta. The prior seasonal effect in cell I2 is multiplied by (1 – Delta). The two terms are summed to reach a smoothed seasonal index for Period 13.
The forecast for the next period is placed in cell J13 with the formula
=G14+H14+I3
totaling the current level, trend, and seasonal index. Notice that although we have just calculated the value of the seasonal index for Period 13 in cell I14, for the purpose of making the current forecast we use the seasonal index from one year back in cell I3.
- The remainder of the time series can now be forecast by selecting cells E14:J14, copying them, and pasting them from E15 to J145. You can get the one-step-ahead forecast by copying G145:J145 into G146:J146. And you can push the final forecast a little further out by following the procedures I suggest in an earlier section of this chapter, “Dealing with the End of the Time Series.”
Starting the Forecasts Earlier
Notice in Figure 5.27 that the forecasting process does not begin until the 13th period. There’s no particular reason that this should be so. Compare Figure 5.27 with Figure 5.28.

Figure 5.28 The forecasts start at t = 1.
In Figure 5.28, I have inserted extra rows for periods –11 through 0. This provides a place to store the seasonal estimates based on the differences between the first year’s mean and its monthly observations. Notice that in Figure 5.28, the first forecast is for t = 1, whereas the first forecast is for t = 13 in Figure 5.27.
With a good, long baseline such as is available in Box-Jenkins Series G, this difference isn’t cause for much concern. Notice, for example, that the values for the root mean square error are very close to one another in Figures 5.27 and 5.28. And see Figure 5.29 for another sort of comparison.
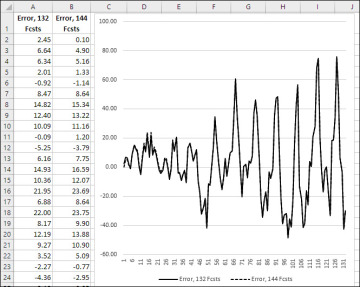
Figure 5.29 The errors are indistinguishable in the chart, but the swings are greater later in both series.
In Figure 5.29, I show the individual errors based on the actual versus forecast differences for time periods 13 through 144; column A shows the errors when the forecasts begin at t = 13, and column B shows the errors when the forecasts begin at t = 1. When the forecasts begin at t = 13, the forecast error for that period is different than when they begin at t = 1. Therefore the level forecast for t = 14, and for subsequent periods, is different for the two sets of forecasts.
In this case the differences between the forecast errors in columns A and B are very small relative to the overall series mean. Nevertheless, the differences are greater during the first 40 to 50 time periods than during the final 40 or 50. You can’t tell that from the chart because the charted values are so close to one another that one charted series obscures the other. But you could easily get a very different result with another set of observations, perhaps one that isn’t so well behaved.
Also, note in the chart that the swings are greater as the level of the series increases. This is an argument for using a multiplicative model rather than an additive model. I discuss the differences between the models in Chapter 7.
First, though, Chapter 6, “Names, Addresses, and Formulas,” discusses some techniques in Excel that can make it much more straightforward to design and interpret the more complex smoothing models.