- What Is Supply Chain Network Design and Why Is It Important?
- Quantitative Data: Why Does Geography Matter?
- Quantitative Data: Why Have Warehouses?
- Quantitative Data: Why Have Multiple Plants?
- Solving the Quantitative Aspects of the Problem Using Optimization
- Data Precision Versus Significance: What Is the Right Level in Modeling?
- Nonquantifiable Data: What Other Factors Need to Be Considered?
- Nonquantifiable Data: What Are the Organizational Challenges?
- Where Are We Going with the Book?
- End-of-Chapter Questions
Data Precision Versus Significance: What Is the Right Level in Modeling?
The discussion about quantitative data brings us to a discussion about data accuracy.
The lessons we learned about significant digits in high-school chemistry will serve us well when doing network design studies.
We learned that measurements were only as accurate as the equipment that took the measurement, and we had to report it as such. And when we combined different measurements together in some equation, our answer could be reported only in terms of the measurement with the least amount of accuracy. This accuracy was expressed in the number of significant digits. For example, if we took one measurement and it was 3.2 units and added that to another measurement of 4.1578 units and added them together, our calculator would give us 7.3578 units. However, we have to report it as 7.4 units because the final answer can have at most two significant digits. To write it otherwise would give the answer a level of accuracy that just wasn’t true.
We often assume that more precise data is always better. However, as our lessons in significant digits taught us, we can be only as precise as our measurements allow. Keeping this concept in mind will serve you well when you are collecting data for your network design models as well.
The adage about bad data leading to bad results, “garbage in equals garbage out,” can sometimes just confuse. This adage does not mean that data needs to be precisely measured to a certain number of significant digits. It just means that the data has to be good enough for the decisions we are making.
Other problems can result when the precision and detail of the data actually get in the way of making good decisions. The cartoon in Figure 1.1 highlights this point very well.
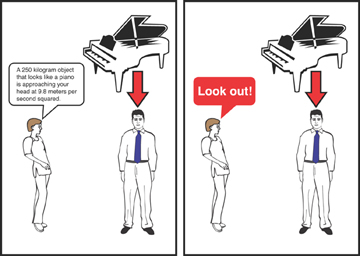
Figure 1.1. Precision Cartoon
In the cartoon, the extra precision on the left actually makes things worse for our poor analyst (who is about to be hit by a piano). The analyst has to spend too much time trying to understand the data and misses the opportunity to take the much-needed action of getting out of the way. In network modeling, our time horizon for making decisions is much longer, but the data is also much more complex. We have seen projects in which the extremely detailed analysis of data causes the project team to miss their opportunity to impact the supply chain in a positive way.
Our goal for collecting data for a network design model is to define the data needed with the right level of significance to make the relevant decisions. Our ultimate responsibility is to report the results with the right level of significance for the organization to make decisions. Our goal is not to ensure that every piece of data is significant to ten decimal places. Therefore it is really a waste of time and often a risk to our project’s success when we report data with more significance than is warranted.
Also keep in mind that when we are running a network design project, we are making decisions about the location of our plants and warehouses to support the business in the future. It is pointless to build a new warehouse to support last year’s business. Although you may start your analysis with historical data as a baseline (we’ll cover baseline in more detail in Chapter 8, “Baselines and Optimal Baselines”), you will eventually want to consider how this data might be different when considering it in a future state. Two specific elements with future uncertainty, for example, include:
- Demand Data—There will be uncertainty in future demand dependent on overall economic conditions, moves by competitors, success of your marketing programs, and so on.
- Transportation Costs—There can be a lot of variance in future prices of transportation dependent on the ever-fluctuating world market price of oil.
Based on the previous, we know our instruments for measuring future demand and transportation cost data are likely going to be inaccurate. Therefore we should consider using fewer significant digits when representing them in the model. If we get push-back to include more significant digits from other stakeholders in the project, we should remind ourselves that if we could accurately predict future demand or the future price of oil, we would benefit more by taking this knowledge to the financial markets.
In Chapter 4, “Alternative Service Levels and Sensitivity Analysis,” we will expand our discussion of this topic to include the use of sensitivity analysis and multiple scenarios to help make our best network design decisions despite this problem of uncertainty. That is, just because we don’t know the future demand or the future price of oil, doesn’t mean we can’t still come up with good solutions.
Often times, the problems we mentioned previously tempt teams into giving up on network design until they get better and more precise data. People get nervous about making decisions without enough significant digits or enough precision. There are two problems with this approach, though:
- You are fooling yourself that you are not making decisions. If you don’t do anything with your supply chain, you are making the decision that there is nothing you can do to improve the supply chain right now. And if you make decisions without any formal data collection and modeling, you are using the resource that is the most imprecise and has the fewest significant digits of all: your intuition.
- You are missing a chance to better understand your supply chain and understand what data you should be collecting. For example, a good practice that we have found is to make initial assumptions, run scenarios, and test those assumptions by varying the data by +/–10%, then +/–20%, and seeing what happens. These runs give you insight into your supply chain and show you the value of the data. For example, if the results are not sensitive to a particular data element, you do not have to spend much time refining that data element as you continue to build out your model.
Of course, when we are looking at the results and output data, we also want to keep our lessons in significant digits in mind. If our significant digits are valid only to the nearest million dollars, then a reported savings of $500,000 should not be considered.
The other concept to keep in mind when analyzing the output comes from introductory statistics. When you first learned about hypothesis testing, you were shown techniques for determining whether two different samples were statistically different from each other. For example, if you ran two marketing campaigns in two different markets and one market came back with average sales of $15,000 per store and the other with $17,500 per store, you could not immediately conclude that the second marketing campaign was better. In general, the higher the variability of the data in the sample size, the harder it is to claim that there is a statistical difference between the two samples.
Although we cannot apply this direct statistical test to our network design models, we can apply the concept. Because we already know that we have a lot of underlying variability in our data (such as future demand, future costs, and so on), we know that we need to show a fairly large savings from the current state to be comfortable with making the decision to implement the recommended change.
Typically, we look for savings of more than 5% to 10% compared to the current situation before we recommend a change. That is, when relocating facilities, if you find savings that reduce costs by 1% to 2% or change services levels by 2% to 3%, we would consider this not statistically different from the current state. When the savings are 15% to 25% however, you can be confident that you have found a statistically different solution.
Of course, you should be careful with this rule. You don’t need to completely disregard the value of relatively small improvements either. The key is to prevent the magnitude of the suggested change from swamping the size of the expected benefit. For example, you might study a $300 million supply chain that is already well rationalized, and only find $250,000 in savings. However, this 0.084% savings might be well worth the bother, if it could be realized by reassigning just two customers. In such a situation, you might think of the $250,000 as being proportionally quite large when compared to the total landed cost of serving these two wayward demand points.
As you go forward in this book and with projects for your company, these are important lessons that will serve you well.