What Is a Deployment Pipeline?
At an abstract level, a deployment pipeline is an automated manifestation of your process for getting software from version control into the hands of your users. Every change to your software goes through a complex process on its way to being released. That process involves building the software, followed by the progress of these builds through multiple stages of testing and deployment. This, in turn, requires collaboration between many individuals, and perhaps several teams. The deployment pipeline models this process, and its incarnation in a continuous integration and release management tool is what allows you to see and control the progress of each change as it moves from version control through various sets of tests and deployments to release to users.
Thus the process modeled by the deployment pipeline, the process of getting software from check-in to release, forms a part of the process of getting a feature from the mind of a customer or user into their hands. The entire process—from concept to cash—can be modeled as a value stream map. A high-level value stream map for the creation of a new product is shown in Figure 5.1.

Figure 5.1 A simple value stream map for a product
This value stream map tells a story. The whole process takes about three and a half months. About two and a half months of that is actual work being done—there are waits between the various stages in the process of getting the software from concept to cash. For example, there is a five-day wait between the development team completing work on the first release and the start of the testing process. This might be due to the time it takes to deploy the application to a production-like environment, for example. As an aside, it has been left deliberately unclear in this diagram whether or not this product is being developed in an iterative way. In an iterative process, you'd expect to see the development process itself consist of several iterations which include testing and showcasing. The whole process from discovery to release would also be repeated many times1
Creating a value stream map can be a low-tech process. In Mary and Tom Poppendieck's classic, Lean Software Development: An Agile Toolkit, they describe it as follows.
- With a pencil and pad in hand, go to the place where a customer request comes into your organization. You goal is to draw a chart of the average customer request, from arrival to completion. Working with the people involved in each activity, you sketch all the process steps necessary to fill the request, as well as the average amount of time that a request spends in each step. At the bottom of the map, draw a timeline that shows how much time the request spends in value-adding activities and how much in waiting states and non-value-adding activities.
If you were interested in doing some organizational transformation work to improve the process, you would need to go into even more detail and describe who is responsible for which part of the process, what subprocesses occur in exceptional conditions, who approves the hand-offs, what resources are required, what the organizational reporting structures are, and so forth. However, that's not necessary for our discussion here. For more details on this, consult Mary and Tom Poppendieck's book Implementing Lean Software Development: From Concept to Cash.
The part of the value stream we discuss in this book is the one that goes from development through to release. These are the shaded boxes in the value stream in Figure 5.1. One key difference of this part of the value stream is that builds pass through it many times on their way to release. In fact, one way to understand the deployment pipeline and how changes move through it is to visualize it as a sequence diagram,2 as shown in Figure 5.2.
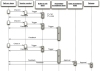
Figure 5.2 Changes moving through the deployment pipeline
Notice that the input to the pipeline is a particular revision in version control. Every change creates a build that will, rather like some mythical hero, pass through a sequence of tests of, and challenges to, its viability as a production release. This process of a sequence of test stages, each evaluating the build from a different perspective, is begun with every commit to the version control system, in the same way as the initiation of a continuous integration process.
As the build passes each test of its fitness, confidence in it increases. Therefore, the resources that we are willing to expend on it increase, which means that the environments the build passes through become progressively more production-like. The objective is to eliminate unfit release candidates as early in the process as we can and get feedback on the root cause of failure to the team as rapidly as possible. To this end, any build that fails a stage in the process will not generally be promoted to the next. These trade-offs are shown in Figure 5.3.
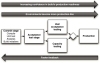
Figure 5.3 Trade-offs in the deployment pipeline
There are some important consequences of applying this pattern. First, you are effectively prevented from releasing into production builds that are not thoroughly tested and found to be fit for their intended purpose. Regression bugs are avoided, especially where urgent fixes need releasing into production (such fixes go through the same process as any other change). In our experience, it is also extremely common for newly released software to break down due to some unforeseen interaction between the components of the system and its environment, for example due to a new network topology or a slight difference in the configuration of a production server. The discipline of the deployment pipeline mitigates this.
Second, when deployment and production release themselves are automated, they are rapid, repeatable, and reliable. It is often so much easier to perform a release once the process is automated that they become "normal" events—meaning that, should you choose, you can perform releases more frequently. This is particularly the case where you are able to step back to an earlier version as well as move forward. When this capability is available, releases are essentially without risk. The worst that can happen is that you find that you have introduced a critical bug—at which point you revert to an earlier version that doesn't contain the bug while you fix the new release offline (see Chapter 10, "Deploying and Releasing Applications").
To achieve this enviable state, we must automate a suite of tests that prove that our release candidates are fit for their purpose. We must also automate deployment to testing, staging, and production environments to remove these manually intensive, error-prone steps. For many systems, other forms of testing and so other stages in the release process are also needed, but the subset that is common to all projects is as follows.
- The commit stage asserts that the system works at the technical level. It compiles, passes a suite of (primarily unit-level) automated tests, and runs code analysis.
- Automated acceptance test stages assert that the system works at the functional and nonfunctional level, that behaviorally it meets the needs of its users and the specifications of the customer.
- Manual test stages assert that the system is usable and fulfills its requirements, detect any defects not caught by automated tests, and verify that it provides value to its users. These stages might typically include exploratory testing environments, integration environments, and UAT (user acceptance testing).
- Release stage delivers the system to users, either as packaged software or by deploying it into a production or staging environment (a staging environment is a testing environment identical to the production environment).
We refer to these stages, and any additional ones that may be required to model your process for delivering software, as a deployment pipeline. It is also sometimes referred to as a continuous integration pipeline, a build pipeline, a deployment production line, or a living build. Whatever it is called, this is, fundamentally, an automated software delivery process. This is not intended to imply that there is no human interaction with the system through this release process; rather, it ensures that error-prone and complex steps are automated, reliable, and repeatable in execution. In fact, human interaction is increased: The ability to deploy the system at all stages of its development by pressing a button encourages its frequent use by testers, analysts, developers, and (most importantly) users.
A Basic Deployment Pipeline
Figure 5.4 shows a typical deployment pipeline and captures the essence of the approach. Of course, a real pipeline will reflect your project's actual process for delivering software.

Figure 5.4 Basic deployment pipeline
The process starts with the developers committing changes into their version control system. At this point, the continuous integration management system responds to the commit by triggering a new instance of our pipeline. The first (commit) stage of the pipeline compiles the code, runs unit tests, performs code analysis, and creates installers. If the unit tests all pass and the code is up to scratch, we assemble the executable code into binaries and store them in an artifact repository. Modern CI servers provide a facility to store artifacts like these and make them easily accessible both to the users and to the later stages in your pipeline. Alternatively, there are plenty of tools like Nexus and Artifactory which help you manage artifacts. There are other tasks that you might also run as part of the commit stage of your pipeline, such as preparing a test database to use for your acceptance tests. Modern CI servers will let you execute these jobs in parallel on a build grid.
The second stage is typically composed of longer-running automated acceptance tests. Again, your CI server should let you split these tests into suites which can be executed in parallel to increase their speed and give you feedback faster—typically within an hour or two. This stage will be triggered automatically by the successful completion of the first stage in your pipeline.
At this point, the pipeline branches to enable independent deployment of your build to various environments—in this case, UAT (user acceptance testing), capacity testing, and production. Often, you won't want these stages to be automatically triggered by the successful completion of your acceptance test stage. Instead, you'll want your testers or operations team to be able to self-service builds into their environments manually. To facilitate this, you'll need an automated script that performs this deployment. Your testers should be able to see the release candidates available to them as well as their status—which of the previous two stages each build has passed, what were the check-in comments, and any other comments on those builds. They should then be able to press a button to deploy the selected build by running the deployment script in the relevant environment.
The same principle applies to further stages in the pipeline, except that, typically, the various environments you want to be able to deploy to will have different groups of users who "own" these environments and have the ability to self-service deployments to them. For example, your operations team will likely want to be the only one who can approve deployments to production.
Finally, it's important to remember that the purpose of all this is to get feedback as fast as possible. To make the feedback cycle fast, you need to be able to see which build is deployed into which environment, and which stages in your pipeline each build has passed. Figure 5.5 is a screenshot from Go showing what this looks like in practice.
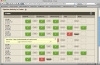
Figure 5.5 Go showing which changes have passed which stages
Notice that you can see every check-in down the side of the page, every stage in the pipeline that each check-in has been through, and whether it passed or failed that stage. Being able to correlate a particular check-in, and hence build, to the stages in the pipeline it has passed through is crucial. It means that if you see a problem in the acceptance tests (for example), you can immediately find out which changes were checked into version control that resulted in the acceptance tests failing.