- Hadoop as a Data Lake
- The Hadoop Distributed File System (HDFS)
- Direct File Transfer to Hadoop HDFS
- Importing Data from Files into Hive Tables
- Importing Data into Hive Tables Using Spark
- Using Apache Sqoop to Acquire Relational Data
- Using Apache Flume to Acquire Data Streams
- Manage Hadoop Work and Data Flows with Apache Oozie
- Apache Falcon
- What's Next in Data Ingestion?
- Summary
Using Apache Flume to Acquire Data Streams
In addition to structured data in databases, another common source of data is log files, which usually come in the form of continuous (streaming) incremental files often from multiple source machines. In order to use this type of data for data science with Hadoop, we need a way to ingest such data into HDFS.
Apache Flume is designed to collect, transport, and store data streams into HDFS. Often data transport involves a number of Flume agents that may traverse a series of machines and locations. Flume is often used for log files, social-media-generated data, email messages, and pretty much any continuous data source.
As shown in Figure 4.4, a Flume agent is composed of three components:
Source—The source component receives data and sends it to a channel. It can send the data to more than one channel. The input data can be from a real-time source (e.g. web log) or another Flume agent.
Channel—A channel is a data queue that forwards the source data to the sink destination. It can be thought of as a buffer that manages input (source) and output (sink) flow rates.
Sink—The sink delivers data to destinations such as HDFS, a local file, or another Flume agent.
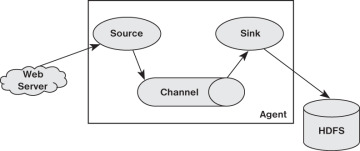
Figure 4.4 Flume Agent with Source, Channel, and Sink.
A Flume agent can have multiple sources, channels, and sinks but must have at least one of each of the three components defined. Sources can write to multiple channels, but a sink can only take data from a single channel. Data written to a channel remain in the channel until a sink removes the data. By default, the data in a channel is kept in memory but optionally may be stored on disk to prevent data loss in the event of a network failure.
As shown in Figure 4.5, Flume agents may be placed in a pipeline. This configuration is normally used when data is collected on one machine (e.g., a web server) and sent to another machine that has access to HDFS.

Figure 4.5 Pipeline created by connecting Flume agents.
In a Flume pipeline, the sink from one agent is connected to the source of another. The data transfer format normally used by Flume is called Apache Avro4 and provides several useful features. First, Avro is a data serialization/deserialization system that uses a compact binary format. The schema is sent as part of the data exchange and is defined using JavaScript Object Notation (JSON). Avro also uses remote procedure calls (RPC) to send data. That is, an Avro sink will contact an Avro source to send data.
Another useful Flume configuration is shown in Figure 4.6. In this configuration, Flume is used to consolidate several data sources before committing them to HDFS.

Figure 4.6 A Flume consolidation network.
There are many possible ways to construct Flume transport networks.
The full scope of Flume functionality is beyond the scope of this book, and there are many additional features in Flume such as plug-ins and interceptors that can enhance Flume pipelines. For more information and example configurations, please see the Flume Users Guide at https://flume.apache.org/FlumeUserGuide.html.
Using Flume: A Web Log Example Overview
In this example web logs from the local machine will be placed into HDFS using Flume. This example is easily modified to use other web logs from different machines. The full source code and further implementation notes are available from the book web page in Appendix A, “Book Web Page and Code Download.” Two files are needed to configure Flume. (See the sidebar “Flume Configuration Files.”)
web-server-target-agent.conf—The target Flume agent that writes the data to HDFS
web-server-source-agent.conf—The source Flume agent that captures the web log data
The web log is also mirrored on the local file system by the agent that writes to HDFS.
To run the example, create the directory as root.
# mkdir /var/log/flume-hdfs # chown hdfs:hadoop /var/log/flume-hdfs/
Next, as user hdfs, make a Flume data directory in HDFS.
$ hdfs dfs -mkdir /user/hdfs/flume-channel/
Now that the data directories are created, the Flume target agent can be started (as user hdfs).
$ flume-ng agent -c conf -f web-server-target-agent.conf -n collector
This agent writes the data into HDFS and should be started before the source agent. (The source reads the web logs.)
The source agent can be started as root, which will start to feed the web log data to the target agent. Note that the source agent can be on another machine:
# flume-ng agent -c conf -f web-server-source-agent.conf -n source_agent
To see if Flume is working, check the local log by using tail. Also check to make sure the flume-ng agents are not reporting any errors (filename will vary).
$ tail -f /var/log/flume-hdfs/1430164482581-1
The contents of the local log under flume-hdfs should be identical to that written into HDFS. The file can be inspected using the hdfs –tail command. (filename will vary). Note, while running Flume, the most recent file in HDFS may have a .tmp appended to it. The .tmp indicates that the file is still being written by Flume. The target agent can be configured to write the file (and start another .tmp file) by setting some or all of the rollCount, rollSize, rollInterval, idleTimeout, and batchSize options in the configuration file.
$ hdfs dfs -tail flume-channel/apache_access_combined/150427/FlumeData. 1430164801381
Both files should have the same data in them. For instance, the preceding example had the following in both files:
10.0.0.1 - - [27/Apr/2015:16:04:21 -0400] "GET /ambarinagios/nagios/nagios_alerts .php?q1=alerts&alert_type=all HTTP/1.1" 200 30801 "-" "Java/1.7.0_65" 10.0.0.1 - - [27/Apr/2015:16:04:25 -0400] "POST /cgi-bin/rrd.py HTTP/1.1" 200 784 "-" "Java/1.7.0_65" 10.0.0.1 - - [27/Apr/2015:16:04:25 -0400] "POST /cgi-bin/rrd.py HTTP/1.1" 200 508 "-" "Java/1.7.0_65"
Both the target and source file can be modified to suit your system.