- One Global Histogram—and Contention
- Per-Block Histograms
- Privatized (Per Thread) Histograms
- Performance
- Conclusion
- Notes
Privatized (Per Thread) Histograms
We've explored CUDA implementations that operate on one histogram per grid (the output histogram, which we operate on with global atomics) and one histogram per block (which we operate on with shared memory atomics). An even finer granularity—one histogram per thread—is a natural next step to explore, and in fact is the only viable way to parallelize histograms on multicore CPUs, since atomic additions are so expensive compared to incrementing per-thread histogram elements that are in each CPU core's L1 cache. A CPU-optimized, multithreaded implementation would spawn M CPU threads for each of the M cores on the system, decompose the problem into M chunks, and compute a histogram for each using a fork/join idiom, and then combine them into a single histogram at the end. When there is one histogram per thread, the intermediate histograms are known in the literature as "privatized" histograms, presumably because each is private to a thread, and not because they are scheduled for divestment by the government.
The CUDA Handbook's source code on GitHub includes a multithreaded implementation of the CPU code. For sufficiently large N, performance should scale linearly in the number of CPU cores. On the EC2 instance type CC1 (which contains two quad-core Intel E5570 processors), the multithreaded version runs at 4.0 Gpix/s, 4.4x faster than the single-threaded version.
Since privatized, per-thread histograms work well on CPUs, might they also work well in CUDA? The goal is less data-dependent performance; many applications would give up some performance to the per-block or per-grid formulations in exchange for not suffering an order-of-magnitude performance degradation for degenerate inputs.
In CUDA, when allocating one histogram per thread, shared memory is the logical choice, since the registers cannot be referenced by index. But because each SM has at most 48KB of shared memory, the number of threads per block is limited even if the developer uses short integers to hold the histogram elements. For example, with 8-bit histogram elements, 64-thread blocks each use 256*64=16,384 bytes of shared memory; that's just a bit too much for SM 1.x class hardware, which reserves 256 bytes of the 16KB of shared memory for parameter passing. SM 2.0 and later hardware then becomes limited to 3 blocks per SM, making for low occupancy. (One positive side effect is that developers can use many registers per thread without further reducing occupancy.)
Layout Considerations
The 256*NumThreads elements of the privatized histograms may be laid out in shared memory in any number of ways. Figure 4 and 5 show two possibilities: one histogram per row (each thread operates on its own row), and one histogram element per row (each thread operates on its own column). These layouts both suffer from poor performance due to using 8-bit memory operands in shared memory.
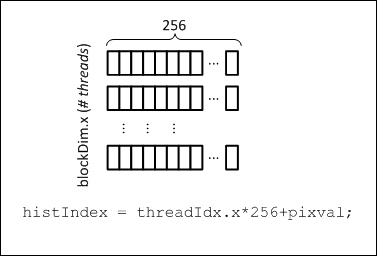
Figure 4 Histogram per row.

Figure 5 Histogram per column.
In the case of degenerate inputs, the layout of Figure 5 causes the threads to reference adjacent shared memory locations. If they were 32-bit memory locations, this layout would have good performance in the degenerate case by minimizing shared memory bank conflicts. We can get the best of both worlds (32-bit memory operations and adjacency in the case of degenerate data) by using the hybrid layout shown in Figure 6: 64 rows that each contain an interleaved set of four packed 8-bit counters, one per thread. If we happen to use 64 threads per block, the array of 32-bit integers coincidentally will be square (64*64).

Figure 6 Histogram per column (interleaved).
Listing 5 shows a device function that increments a privatized histogram element using the layout of Figure 6. This operation uses 32-bit shared memory accesses and minimizes bank conflicts for completely uniform data, in which case the threads increment adjacent 32-bit shared memory locations. As a result, performance is less likely to degrade due to contention.
Listing 5Incrementing a 32-bit, privatized histogram element.
inline __device__ void incPacked32Element( unsigned char pixval ) { extern __shared__ unsigned int privHist[]; const int blockDimx = 64; unsigned int increment = 1<<8*(pixval&3); int index = pixval>>2; privHist[index*blockDimx+threadIdx.x] += increment; }
Block-Wide Reduction
Once the privatized histograms have been accumulated in shared memory, a myriad of ways are available to compute the reduction of each histogram element and add it to the global memory output. Listing 6 shows one operation that they share in common: splitting the four packed 8-bit partial sums into two pairs of packed 16-bit partial sums. Up to 256 such partial sums can be added together before risking overflow; since we have one histogram per thread, overflow can only occur if we use at least 256 threads per block. On the other hand, using so many threads is unlikely because the number of threads is limited by the small amount of shared memory per SM.
Listing 6Unpacking 8-bit histogram elements into 16-bit histogram elements.
unsigned int myValue = privHist[i*64+threadIdx.x]; int sum02, sum13; sum02 = myValue & 0xff00ff; myValue >>= 8; sum13 = myValue & 0xff00ff;
For a thread count of 64, each row of the array of privatized histograms contains 64 columns, each of which is a 32-bit value that can be unpacked per Listing 6. It's tempting to perform a reduction on each row and emit the four resulting sums into the output histogram; but that approach would not be work-efficient. Instead, we can have each thread compute the sum of a row in a simple loop. Listing 7 gives the implementation for 64-thread blocks: Each thread computes two 32-bit sums, each of which contains two packed 16-bit partial sums computed per Listing 6. The code of Listing 7 performs exactly 256 atomic operations, one for each output histogram element.
Listing 7Per-block reduction of privatized histograms.
template<bool bClear> __device__ void merge64HistogramsToOutput( unsigned int *pHist ) { extern __shared__ unsigned int privHist[]; unsigned int sum02 = 0; unsigned int sum13 = 0; for ( int i = 0; i < 64; i++ ) { int index = (i+threadIdx.x)&63; unsigned int myValue = privHist[threadIdx.x*64+index]; if ( bClear ) privHist[threadIdx.x*64+index] = 0; sum02 += myValue & 0xff00ff; myValue >>= 8; sum13 += myValue & 0xff00ff; } atomicAdd( &pHist[threadIdx.x*4+0], sum02&0xffff ); sum02 >>= 16; atomicAdd( &pHist[threadIdx.x*4+2], sum02 ); atomicAdd( &pHist[threadIdx.x*4+1], sum13&0xffff ); sum13 >>= 16; atomicAdd( &pHist[threadIdx.x*4+3], sum13 ); }
Managing Overflow
On its own, the code we've discussed will not work correctly if any thread increments the same histogram element more than 256 times. To avoid overflow, three basic strategies can be employed:
- Overflow check. Check for overflow after incrementing the histogram element, and fire an atomic into either shared memory or global memory.
- Overflow avoidance. Ensure that no thread considers more inputs than any single histogram element can accommodate.
- Periodic merge. Periodically merge the privatized histograms into the output histogram and zero them out.
The overflow check strategy turned out to be too slow—checking for overflow for every input element introduced too much overhead into the inner loop.
The second strategy, overflow avoidance, is easy to implement because the number of input elements considered by each thread is proportional to the number of thread blocks in the kernel launch. If w*h is the number of pixels and numthreads is the number of threads per block, we can write the following:
int numblocks = INTDIVIDE_CEILING( w*h, numthreads*255 );
and no thread will consider more than 255 elements. [4] If the inner loop is unrolled as previously described, the number of blocks must be increased accordingly:
int numblocks = INTDIVIDE_CEILING( w*h, numthreads*(255/4) );
The disadvantage of overflow avoidance is that it can be too conservative: Every block must merge its privatized histograms into the final output, and that extra effort is wasted if very few histogram elements ran the risk of overflow.
It turns out that periodically merging the privatized histograms into the output, just often enough to prevent any histogram element from overflowing, was measurably faster than avoiding overflow by launching more thread blocks. The kernel given in Listing 8 implements this strategy if the template parameter bPeriodicMerge is true.
Listing 8Privatized histogram implementation.
template<bool bPeriodicMerge> __global__ void histogram1DPerThread4x64( unsigned int *pHist, const unsigned char *base, size_t N ) { extern __shared__ unsigned int privHist[]; const int blockDimx = 64; if ( blockDim.x != blockDimx ) return; for ( int i = threadIdx.x; i < 64*blockDimx; i += blockDimx ) { privHist[i] = 0; } __syncthreads(); int cIterations = 0; for ( int i = blockIdx.x*blockDimx+threadIdx.x; i < N/4; i += blockDimx*gridDim.x ) { unsigned int value = ((unsigned int *) base)[i]; incPrivatized32Element( value & 0xff ); value >>= 8; incPrivatized32Element( value & 0xff ); value >>= 8; incPrivatized32Element( value & 0xff ); value >>= 8; incPrivatized32Element( value ); cIterations += 1; if ( bPeriodicMerge && cIterations>=252/4 ) { cIterations = 0; __syncthreads(); merge64HistogramsToOutput<true>( pHist ); } } __syncthreads(); merge64HistogramsToOutput<false>( pHist ); }