- Design Principles
- Stacked Bar Charts
- Waterfall Charts
- Time Series Charts
- Bivariate (X-Y) Charts
- Small Multiples
- Two-by-Two Matrices
- Period-Share Chart
- Pareto Charts
- Tables
- Treemaps
- Thinking Like a Cannibal: The Case for Redrawing
- Summary
Thinking Like a Cannibal: The Case for Redrawing
Good visualization flows naturally from good design. And good design results from clear thinking about desired objectives; the format should always strive to answer key questions. Analysts should, on a regular basis, revisit existing exhibits to determine whether the questions they answer are the right ones. Equally important, analysts should ask themselves whether the design, format, and details of existing reports answer those questions as well as they could. When an exhibit falls short, consider revisioning and redrawing it.
Redrawing an existing exhibit format requires a certain amount of courage, particularly when an existing process or shipping product depends on it. Let us try an example or two.
A Patch Job for Ecora
Ecora Patch Manager's Reporting Center produces a patch-management status chart14 and associated table that summarizes the effectiveness of the patch application process (see Figure 6-29). The chart shows the number of patches available, plus the total number available, and groups these statistics according to the severity of the patch. Unfortunately, the chart does not tell us much other than aggregate statistics, and the exact question it answers seems vague. Questions this exhibit should answer include these:
- What patches are the most troublesome (hardest to apply)?
- How effective or efficient is the patch management solution overall?
- How large is the window of exposure for systems that have not yet applied patches?
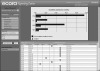
Figure 6-29 Redrawing Candidate: Ecora Patch Manager
Copyright © 2005 Ecora Corporation. Reprinted with permission; all rights reserved.
We can tell that the chart is not doing its job well just by looking at it. The dead give-away is that two bars use the same unit of measure (one for total number of patches available, and one for installed patches). Bar charts are typically good candidates for redrawing using a bivariate chart.
Redrawing this chart using two variables improves matters somewhat. Let us assume that effectiveness, or lack thereof, matters most. Therefore, the chart ought not to emphasize applied patches, but missing patches—in particular, the percentage of missing patches. Assume for the moment that the number of overall required patches matters to the reader, too, because it implies the overall effort required to apply them. Therefore, the two axes show the number of available patches (on the x-axis) and the percentage of these that were not installed (on the y).
An initial redrawing of the exhibit results in the example shown in Figure 6-30. Notice the change in terminology from "available" patches to "required," which better reflects the mandatory nature of patch management; we want available patches to be installed, don't we?
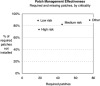
Figure 6-30 Ecora Patch Manager Exhibit (Redrawn)
Although this bivariate chart improves on the original, it still presents problems: does the reader care about the number of required patches in aggregate? Probably not. The chart becomes more relevant when expressing the number of required patches on a per-machine basis. For the sake of simplicity, assume that the number of affected machines in the sample is five. Figure 6-31 shows the second revision for the chart.
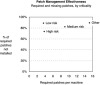
Figure 6-31 Ecora Patch Manager Exhibit (Second Redrawing)
The new chart improves on the two previous iterations, but we are not done yet. Examining the data presented in the table below the original Ecora graph, we notice additional data that might make the exhibit even more relevant: per-patch performance data, expressed in terms of the minimum, maximum, and average days required to apply each patch. A stock-chart style exhibit provides an ideal way to express this concept; a secondary table underneath provides the per-machine missing patch statistics.
Figure 6-32 contains the final redrawn patch management exhibit (using hypothetical min/max/average data, since we don't have the actual figures). The crossbars for the average patch time appear as opaque white-colored crossbars, which have the effect of "erasing" part of the hi-low bars.
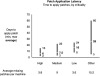
Figure 6-32 Ecora Patch Manager Exhibit (Final Redrawing)
All in all, the final exhibit answers our key questions much better than the original. It shows the average, minimum, and maximum patch latency metrics for four classes of patches, as well as the average number of missing patches per machine in a mini-table below the chart. The revised chart contains twice as many data points as the original, and the data are more revealing. The vertical axes show mean "per patch" and "per machine" performance statistics instead of simpleminded, non-normalized raw numbers. And aesthetically speaking, the new chart's relatively unadorned, functional format focuses the viewer's eye on the data.
The revision process revealed four lessons. Analysts should always:
- Question the exhibit format when the complexity of the underlying message exceeds the chart's ability to communicate it faithfully.
- Dig deeper for richer, more relevant data to answer key questions.
- Consider nontraditional formats, such as our "stock chart" adaptation.
- Use iterative revisions to zero in on the right design for the exhibit.
Reorienting SecurCompass
It seems safe to assume that no security analyst would intentionally create a bad graphic. But sometimes reality challenges even the most cherished assumptions. Consider the "benchmarking" chart shown in Figure 6-33, created using a security assessment tool from Espiria called SecurCompass®. The exhibit, which appeared in the Computer Security Journal, shows the average score (and ranges) by industry for security compliance.15 But you would never know it—the chart is literally incomprehensible:
- Nondescript title: The title "Industry Benchmarks" sounds terrific, but what is the "benchmark," and how was it derived?
- Poor series labeling: The words "low" and "high" suggest relative measures—but relative to what?
- Poor axis labeling: What unit of measure does the vertical axis represent?
- Mysterious methodology: From whom was the data obtained, and over which periods?
- Obnoxious formatting: Readers must crane their necks sideways to read the category labels, and the data series bars (formatted in 3-D, naturally) seem far too narrow.
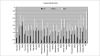
Figure 6-33 Redrawing Candidate: SecurCompass
Copyright © 2005 CMP Media. Reprinted with permission; all rights reserved.
This exhibit is a real howler. Ironically, the authors of the exhibit actually have interesting data to present. For example, in the narrative support immediately preceding the exhibit, the reader learns that the "low" and "high" data points refer to the minimum and maximum scores observed in each industry. Four pages prior to that, the reader learns that the numeric scale (0 to 5) refers to scores on a self-assessed security compliance survey. Most significant, the first page of the report—thirteen pages before the exhibit—implies that the sample size for the study exceeded 350 organizations. All these details matter, and should have appeared in the exhibit. It is almost as if the authors were ashamed of their good (?) work, and wanted to bury the evidence.
Cleaning up the SecurCompass exhibit requires a change in format. A "hi-lo-close" stock chart gives us an effective way to show the minimum/maximum/average data points. Changing the format to vertical permits the viewer to read all the industry labels. A few additional chart tweaks help, too:
- More honest chart title (these are scores for self-assessment, not benchmarks)
- Inclusion of sample size (350 companies) and sample interval (2001 to 2005)
- A clearer label for the dependent axis ("mean compliance score and range," plus some minimal examples)
- Data sorted by mean industry score
- Softened grid lines
Figure 6-34 shows the redrawn exhibit. Look at the difference. The headline pops right out. Banks and financial services companies score highest, health care and automotive the lowest. The data also suggest that some industry scores vary more than others; compare insurance, for example, with high tech.
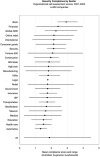
Figure 6-34 SecurCompass Exhibit (Redrawn)
Although redrawing the exhibit provides much more immediacy and impact, it also highlights potential shortcomings in the data. For instance, why do the legal scores vary so little? (Likely answer: a very small sample.) In addition, the total number of companies in the study (more than 350) seems low compared to the number of industries (25, which equals a mean 14 samples per industry). It would have been better to condense the industries, perhaps by half. Increased sample density would have made it possible to include additional statistical information like standard deviations or quartile ranges—crucial for understanding variances within industries.
Finally, readers will note that I have changed the title to de-emphasize benchmarking. There is a simple reason for this. Benchmarks imply point-in-time measures, but the sample interval covers a relatively long period of time (four years). Security practices change quickly; scores averaged over a four-year period cannot serve as credible "benchmarks"—although one-year averages can. The alternative title I supplied ("Organizational self-assessment scores, 2001-2005) is more honest and sidesteps the benchmarking issue.
The SecurCompass example teaches several lessons:
- Charts should explain themselves. If the reader can't figure out a chart without reading the surrounding narrative, it is a bad chart.
- Not all data points require labels. For charts whose primary function is to compare cross sections, simply sorting the data works better than labeling every point.
- Good charts never "bury the lead." If the interesting data from the chart aren't intuitively obvious, redraw the chart.
Managing Threats to Readability
The final candidate for vivisection in this chapter is Symantec's DeepSight Threat Management System (TMS). The service aggregates and summarizes a wealth of information about Internet-based threats gathered from distributed sensors across the globe. One of the tabs on the management console labeled "Firewall Statistics" shows the most popular network ports subjected to hostile probing. Figure 6-35 shows a sample screen capture taken during the spring of 2005. Nice-looking graphics—but wait! Where is the legend that tells us what the colors on the horizontal bars mean?
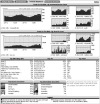
Figure 6-35 Symantec Threat Management System
Copyright © 2005 Symantec Corporation. Reprinted with permission; all rights reserved.
Oh, there it is—at the very bottom of the web page, accessible only after scrolling past several completely unrelated tables. Although it is not a fatal flaw, it certainly detracts from readability, because it requires readers to move their heads back and forth between the exhibit (at the top) and the legend (at the bottom).
So what is wrong with this exhibit? Nothing fatal; most readers will find the exhibit—which appears to be a variant of a small multiple—to be perfectly adequate. But upon closer inspection, certain qualities of this exhibit seem odd:
- Why the extra prominence given to the leftmost graphics? Do they summarize the four (times two) small multiples to the right? (Answer: The leftmost graphics are not summaries. They are larger merely because they show the number-one ranked ports.)
- Corollary question: does the extra width of the leftmost graphic signify anything important? (Answer: No.)
- Why don't the legend keys seem to make much sense? What does "Volume of sensors or source IPs observed . . ." mean? (Answer: It is poorly worded.)
- Some of the graphs on the right side of the ". . . By Increased Sensor Count" exhibit, upon first glance, show volumes that look higher than the leftmost graphs. Is this accurate? (Answer: No. The vertical scales are different, which distorts the visual impression. The scale for the graph of the #1 port is a ridiculous eight times bigger than #5.)
- The red horizontal lines indicate port probe volumes three standard deviations above the seven-day trailing average. Is that an appropriate threshold? (Answer: Probably not. Recall from Chapter 5 that for normal distributions, data points within one standard deviation (s) on either side of the mean covers two-thirds of the points. Two standard deviations covers 95%, and three covers 99.7%. The TMS data might be distributed normally; even if it is not, three standard deviations seems excessive.)
Based on the concerns I have expressed regarding format, scaling, and labeling, the reader can probably guess how I chose to fix the exhibit. Figure 6-36 shows a redrawn version that includes these changes:
- The leftmost graphics' size and scale match those on the right.
- All the small multiples' baselines align.
- Vertical axes maintain consistent scales.
- Grid lines vanish.
- The number of standard deviations in the "threshold" line (red) decreases from three to two, and its graphical representation changes to a gray band extending on both sides of the mean.
- Plain-English service names replace the cryptic, IANA-style service names used in the original.
- Chart legend labels vanish; the one relevant legend item (regarding the threshold line) morphs into annotations applied directly to the leftmost multiples.
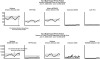
Figure 6-36 Symantec Threat Management System (Redrawn)
The redrawn version communicates the facts more truthfully. Due to the consistent scaling for the y-axis, the reader immediately notices the Pareto-like properties of both the number of detected probes and source IP addresses: after the third port, the numbers drop off sharply. The rescaled version also removes an inadvertent distortion. Instead of an epidemic of traceroute attacks (top row, #4), one sees that the number of detected probes for this port is in fact extremely low relative to the top three.
In addition, the combination of the tightened "threshold line" from three standard deviations to one, and its corresponding extension to below the mean, yields an interesting insight. Most of the time, the levels of probe traffic seem to fall within fairly predictable bands.
The redrawn exhibit could arguably benefit from further improvements. To keep things simple, I omitted time markers on the horizontal axes. Were this exhibit used for forensics rather than (as I imagined) a heads-up dashboard, it would require x-axis markers. And form factor of the exhibit may seem excessively horizontal.
Symantec's Threat Management System provides valuable information, but its slapdash execution makes it less effective than it could be. Its mistakes teach us several lessons:
- To minimize distortions, small multiples should maintain consistent scales for the x- and y-axes.
- Overly wide anomaly thresholds (more than two standard deviations) may not provide enough visual cues to allow viewers to spot unusual behavior. Narrower bands (one or two standard deviations) may provide more value.
- Chart legends should stay as close to the point of use (the charts!) as possible. If possible, consider eliminating the legend in favor of on-chart annotations.
- Superfluous data merely adds clutter. Remove an extra data series not directly related to the main point of the exhibit.
- Small-multiple titles work better when expressed in plain English.