- Business Problem
- Solution Overview
- Data Model
- Technical Solution
- Managing the Solution
- Next Steps
- Summary
Solution Overview
We will build an extraction, transformation, and loading (ETL) process to add the Web site's visit-tracking data to the corporate data warehouse. We will use the data mining features of Analysis Services to help discover patterns in this data and provide the information back to the business.
Business Requirements
The high-level requirements to support the business objectives are as follows:
-
Customer segmentation. The data warehouse already has excellent profiling information on customers that is obtained through a popular store loyalty card program. This information includes demographic profiles and detailed purchasing histories, because the customer's unique card number can be used to identify store transactions. However, the business also needs a profile of customers' online activities.
The main areas of interest are frequency, or how often the customer uses the Web site, and recency, or how much time has elapsed since they visited the site. There is already information in the data warehouse on the third area of interest, which is intensity, or how much money the customer is spending through the Internet channel.
When these Internet profiling attributes are available, customers can be segmented into groups with relatively similar behavior. Analysts can use the information for marketing purposes, such as producing lists of customers for direct mail campaigns, as well as performing further analysis using the attributes and groups that we identified.
- Online recommendations. They would like to add an online recommendations feature to the new DVD area of the Web site to drive additional profit per online transaction. When a customer adds a DVD to her shopping basket, she must be prompted with a short list of other titles that she may be interested in.The performance of this recommendation needs to be good because any delay in the responsiveness of the Web site has been shown to lead to more abandoned transactions. Also, the recommendation must include items sold through the physical stores as well as the Web site, because the stores currently make up the bulk of the sales.
High-Level Architecture
We will add the Internet visit information to the existing data warehouse and Analysis Services cubes. Because the e-commerce application already extracts data from the Web logs and inserts it into a relational database, we will use this as the source for the ETL process. The data in this source database already has discrete user sessions identified.
Many e-commerce applications (including those based on the Microsoft Commerce Server platform) provide this kind of extraction and log processing functionality, but for custom Web sites, the only available tracking information may be the raw Internet Information Server (IIS) logs. A full treatment of the steps to extract this kind of information from Web log files is beyond the scope of this chapter; see the sidebar "Extracting Information from IIS Logs" for a high-level explanation.
After this information is in the data warehouse, we will use the data mining features of Analysis Services to achieve the business goals for segmentation and recommendations, as shown in Figure 10-1. For each area, we will create a data mining structure that describes the underlying business problem and then run the appropriate data mining algorithm against the data to build a mathematical model. This model can then be used both for predictions such as recommending a list of products or for grouping information in cubes together in new ways to enable more complex analyses.
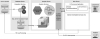
Figure 10-1 High-level architecture
Data mining in Analysis Services has several different types of algorithms to perform tasks such as classification, regression, and segmentation. We will use the Microsoft Clustering algorithm to create a customer segmentation mining model, and then the model will provide these categories of customers' online behavior as a new dimension for cube analysis. We will use the Microsoft Association algorithm to build a data mining model that can be used to make product recommendations, and then add code to the Web site to query this model to suggest appropriate DVDs for online shoppers.
Business Benefits
The solution will deliver the following benefits:
- Targeting direct mail and other marketing to identified groups of customers that will probably use the Internet channel will decrease the overall cost of marketing and increase the company's market share.
- Profitability will be improved by increasing the average number of items sold per transaction, such as selling more DVDs in one transaction and still incurring one shipping cost.